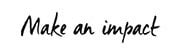
By Riccardo Rebonato, Scientific Director, EDHEC-Risk Climate Impact Institute; Professor of Finance, EDHEC Business School
This article by Riccardo Rebonato, Scientific Director of EDHEC-Risk Climate, has been originally published in the February newsletter of the Institute. To subscribe to this complimentary newsletter, please contact: [email protected].
EDHEC-Risk Climate Impact Institute Scientific Director Riccardo Rebonato offers a concise overview of the key findings from the foundational White Paper from our research programme on modelling the impact of climate change on asset prices. The article delves into the crucial role of scenario analysis and stress testing in grasping the economic consequences of climate change on asset valuation. It explains how we enhance the powerful IPCC framework with probabilistic data to improve its relevance for risk and investment practitioners. It shows how empirical analysis is used to identify strong relationships between economic, demographic, and technological variables and how we derive valuable insights into the likelihood of various climate outcomes, including extreme events. By offering distributions of climate scenarios, such as temperature variations, damages, and cash flow impairments, this novel framework equips financial decision-makers with vital tools to effectively manage climate-related risks.
Knowing which economic consequences climate change will have for asset pricing is clearly important for financial decision-makers. One way to present this information is via scenario analysis and stress testing. Scenario analysis is usually defined as the exploration of ‘plausible’ outcomes, where the term ‘plausible’ clearly has an implicit probabilistic connotation, which is, however, usually not quantified. It is not limited to the analysis of the most likely outcome, but does not usually look at extreme conditions. As for stress testing, the term refers to the scenarios that are still plausible, but also in some sense ‘extreme’. The two requirements (being extreme yet plausible) clearly generate a tension. Despite these differences in ‘intensity’, scenario planning and stress testing are methodologically identical, and they differ only in the likelihood of the events they consider. This methodological equivalence is important, because, as we shall discuss, our goal is to provide investors with a full distribution of outcomes – and when this is available, then the qualitative distinction between scenario analysis and stress testing becomes irrelevant – as the user now has full visibility of the relative likelihood of all the possible outcomes, and can therefore interrogate both the body and the tails of the distribution.
The use of stress testing and scenario analysis is a generally accepted concept in financial risk management.[1] However, climate scenarios are different in two respects: because they cannot be built by ‘resampling’ past history (as we have not encountered yet instances of the climate damages whose effects we want to explore); and because the end users of the scenarios are unlikely to be able to associate them even with very approximate probabilities (again, this is due to the unprecedented nature of climate change in historical times). In this paper, we therefore propose a framework to produce scenarios that reflect the full uncertainty of outcomes, and give an (approximate) assessment of the relative likelihood of their occurrence.
A substantial body of high-quality work has already been devoted to creating climate scenarios (the IPCC framework). These scenarios, however, were not designed with financial decision-makers in mind, and therefore are not well suited to their needs. In particular, the IPCC scenarios:
Cognisant of the fact that these IPCC scenarios have acquired quasi-benchmark status, we have pursued a two-pronged approach in our attempt to provide financial decisionmakers with scenarios that better fit their purpose. With the first strand, we have strived to remain as close as possible to the established IPCC approach, but to add a probabilistic imension to the resulting climate outcomes. By ‘remaining as close as possible’ we mean that we have reverse-engineered and assumed to be correct the relationships between economic, demographic and technological variables implied by the IPCC models. These relationships are highly stylised (linear regressions) and sometimes questionable, but, in our first strand, we have made the choice to accept them in toto for the sake of consistency and comparability with the results of the IPCC scenarios.
With our second approach we have taken a bolder departure from the IPCC framework, and we have recovered the required relationships between economic, demographic and technological variables from careful empirical analysis (that allows for highly non-linear dependencies), rather then from the simplified linear models assumed in the IPCC framework. Three powerful empirical relationships among the state variables that we have uncovered have greatly helped us in the task. Simplifying considerably, these are our findings.
The power of these three relationships is that, for instance, given a carbon-tax trajectory, we can work out the resulting emissions and temperatures; or that, conversely, given average emission-reduction speed (that could come from a variety of different abatement patterns) we can estimate the associated carbon tax (and express an opinion on its feasibility or likelihood).
Thanks to these relationships, we want to provide the financial decision-maker with distribution probabilities of climate outcomes – of temperatures, damages, and, ultimately, impairments to cashflows. These probabilities can be conditional or unconditional. For instance, a distribution of, say, temperatures obtained after an emission policy is exogenously assigned becomes conditional on the chosen emission policy, and can be very different from the temperature distribution associated with a different abatement policy. Pursuing this example, the distribution of temperatures would become unconditional if the various conditional distributions were combined and weighted by their probability of occurrence.
Assigning conditional distributions, while still demanding, is simpler than estimating unconditional distributions (because the task of assessing the relative likelihood of different abatement policies can be by-passed). This is probably why, explicitly or implicitly, a conditional approach is more frequently chosen. For instance, the Task Force on Climate-related Financial Disclosures (TCFD) recommends to use (i.e., to condition on) two scenarios (one of which should be Paris-Agreement aligned). The Network for the Greening of the Financial Sector (NGFS) offers scenarios that are conditioned both on the ‘Middle of Road’ IPCC narrative, or SSP (for Shared Socioeconomic Pathway – a description of possible technological, demographic and economic pathways for the twenty-first century) and on four approximate Representative Carbon Pathways (RCPs). And if one embraces the full IPCC approach, then one effectively conditions the results on all the possible feasible combinations of narratives and carbon pathways. To arrive at an unconditional distribution one would need i) to ensure that anything that can happen has been covered; and ii) to assign probabilities to the two TCFD scenarios; to the four NGFS combinations; or the tens of IPCC-scenario combinations.[2]
We recognise that arriving at unconditional distributions is a formidable task, but, exactly because the task is so heavy, it should not rest on the shoulders of a finance professional. This is why our aim is to produce not only conditional, but also unconditional, distributions of climate outcomes. Regarding the latter, we show how, through careful analysis of the fiscal and technological implications of different abatement policies, we can provide useful information in determining the range of the distribution of abatement aggressiveness. But we are also more ambitious: by introducing the new technique of ‘indirect elicitation’ (based on the idea of using information elicited from economists about one variable to obtain information about another, related, variable), we are able to learn a lot about the shape of the distribution of abatement policies.
The final outcome of our analysis is therefore a full distribution (conditional or unconditional) of climate outcomes, that can provide the financial planner with information about the huge dispersion of temperatures and, via the damage function, of damages; with an estimate of which outcomes are more likely, and should therefore attract greater attention; and with an appreciation of what lies in the ‘tails of the damage distribution’ (in the very-low-probability regime, that is, where scenario analysis morphs into stress testing). This has important practical investment implications: for instance, it is enough to translate the dispersion in temperatures into differences in return volatility for assets with different climate-risk exposures to alter the allocations across these assets, even without any changes in expectations. Probabilistic information about damages derived from our approach, however, could also be fed into advanced valuation models to obtain a full distribution of expected prices, from which average, standard deviation, skewness and higher moments can be obtained.
Footnotes
[1] Many international regulators of the banking system, for instance, require that stress testing and scenario analysis should complement statistical measures of risk, such as Value at Risk.
[2] Since in all these cases the first condition – i.e., the spanning of the full range of what can reasonably be expected to happen – is not met, the second becomes irrelevant.