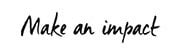
By Gianfranco Gianfrate, Professor of Finance, EDHEC Business School, Research Director, EDHEC-Risk Climate Impact Institute
As climate change and global warming are being addressed by tougher regulations, new emerging technologies, and shifts in consumer behaviours, global investors are increasingly treating climate risks as a key aspect when pricing financial assets and deciding on the allocation of their investment portfolios. Recent estimates are shedding light on the broader indirect impact of climate change on the value of assets held by banks and financial companies. Battiston et al (2017) find that, while direct exposures to the fossil fuel sector are small, the combined exposures to climate policy-relevant sectors are large, heterogeneous, and amplified by large indirect exposures via financial counterparties. Thus, the exposure to climate risk could potentially pose systemic threats to global financial stability.
While the relationship between climate risk exposure and share prices is receiving growing attention from scholars and investors, the impact on corporate bonds and loans appears relatively underexplored. We contribute to filling this gap in the literature by investigating whether a firm’s exposure to climate risks, measured as its level of CO2 emissions and carbon intensity, is associated with Merton’s distance to default, a measure of creditworthiness widely used by rating agencies and investors. Several papers have analysed the influence of sustainability factors either on a firm’s value or on the cost of its debt, while this study focuses on the default probability in terms of distance to default.
Using a panel least squares regression, it is observed that there is a significant and negative relationship between distance to default and the natural logarithm of CO2 emissions, ceteris paribus. We find that this result is robust also when carbon intensity, which is the ratio between carbon emissions and sales, is used. Several robustness checks are performed and the results confirm a significant and negative relationship between distance to default and CO2 footprint.
In order to investigate causality between climate risk exposure and creditworthiness, we investigate the impact of the 2015 Paris Agreement as an exogenous policy shock. After the Paris Agreement, high-emitting companies significantly shorten their distance to default in comparison with low emitters. This finding supports the view that financial markets are increasingly pricing the climate risk exposure of listed companies – especially because of growing commitment among institutional investors (Dyck et al [2019]; Krueger et al [2020]).
All the above-mentioned results not only underline the importance of carbon awareness as a business strategy for polluting firms, but also show the key role it plays with respect to those lenders that are exposed to their clients’ default and reputational risk. However, whether investors consider the level of CO2 emissions in their corporate fixed income investments remains underexplored. Carbon footprint can be measured at absolute level but also in terms of carbon intensity. The latter measure, obtained by scaling total emissions by firm revenue, captures the operational configuration of companies and therefore their ability to switch to less polluting technology.
Credit risk is defined as the risk that a borrower will not be able to meet its financial obligations on time. The Basel Committee defines it as the risk that a borrower will default on debt by failing to make the required payments. Among the approaches used in practice to estimate the probability of corporate default, the structural approach – which calculates the default probability on the basis of the firm’s capital structure – is widely used.
In particular, the distance to default is the number of standard deviations that the firm’s asset value is away from the default and can be defined as:
where
is the is the firm’s asset value at time 0,
are the firm’s value drift rate and volatility,
is the book value of the firm’s liabilities to be paid by time t.
For the empirical analysis, we calculate the one-year probability of default for our sample in the period from 2008 to 2018. In particular, the value of asset V is calculated for each trading day, approximating it as the sum of the market value of equity and book value of liabilities for the same date. Using the obtained series of asset value estimates, log asset returns are calculated and their volatility is then computed. The newly calculated volatility of asset value is introduced to the inverted Black-Scholes formula to obtain a new series of market values of assets, and a new value for
is then computed.
This process is repeated iteratively until the difference between two adjacent asset value estimates (calculated as the sum of squared differences) is lower than a small measure, chosen arbitrarily as 10–5. Once the asset values are obtained, the next step is the calculation of distance to default and corresponding probability of default. The missing value is m, which is calculated as the natural logarithm of the expected returns obtained using the Capital Asset Pricing Model.
Our sample consists of the companies included in the Bloomberg Barclays Agg Corporate index. Out of the index constituents, only companies that issued investment-grade fixed-rate corporate bonds are included: the final sample comprises 458 companies observed from December 2006 to December 2017.
For the calculation of annual distance to default, daily data for market value of equity, index returns and risk-free returns are employed. For liabilities, book values are used, meaning only annual observations were available in most cases. All data are collected from Thomson Reuters DataStream, and expressed in US dollars. All data on emissions are from Asset4.
To test the relationship between distance to default and climate risk exposure, we quantify the carbon footprint – measured as the amount of CO2 emitted – and carbon intensity, measured as the ratio between CO2 emissions and firm revenue.
The control variables are identified in the existing literature as corporate characteristics that appear to influence the distance to default. In particular, these are:
The baseline tests examine the relationship between a firm’s carbon footprint and its distance to default using the following specification:
where the dependent variable is the distance to default of firm i in year t, is the carbon footprint measured either as the amount of CO2 emissions or as carbon intensity obtained as CO2 emissions scaled by firm revenue,
are a set of firm-level, industry and country controls in year t, and
are year fixed effects.
An initial investigation of the data is obtained by partitioning the sample by CO2 emission levels. The pooled data are divided into quintiles, each containing about 453 observations. Quintile 1 contains the top 20% of companies with the lowest level of carbon emissions, and the fifth quintile contains the bottom 20%.
Figure 1 demonstrates that the average annual distance to default decreases as the level of carbon emissions increases. It can be observed that the negative correlation between CO2 emissions and Merton’s distance to default is consistent and is approximately linear.
As a further step, panel least squares regressions are run between distance to default and the natural logarithm of emissions. These regressions allow us to establish if the relationship analysed in the descriptive statistics section is significant.
First, a regression is run with only one explanatory variable, the natural logarithm of total emissions. Even though the adjusted R-squared of the first regression is very low (0.026), the independent variable appears to be significantly and negatively correlated with Merton’s distance to default.
A second regression is then performed mantaining Merton’s distance to default as a dependent variable and the natural logarithm of total emissions as an independent variable, but also including all the control variables described in the section above.
From figure 2, it can be observed that all the variables used are significant at 10%, 5% and 1% significance levels, with the exeption of retained earnings/total assets and working capital/total assets. This second regression has a good explanatory power, with an adjusted R-squared of 30.9%. It can be noted that the natural logarithm of carbon emissions has a highly significant negative relationship with distance to default. Therefore, we can expect companies that produce more CO2 emissions to face higher risks in terms of activity disruptions or payments of fines and, hence, a smaller distance to default. Emissions are a part of non-financial data that is clearly considered by investors when making decisions. In terms of economic significance, an increase by 1% in carbon emissions reduces the firm’s distance to default by about 28.6% on average, all other variables remaining constant. The fact that the adjusted R-squared can be improved leads to the idea that other non-financial variables should be considered in the investment analysis.
All the control variables used are indicators of a company’s high probability of bankruptcy from a financial point of view. The relationship between the distance to default and the debt ratio is negative and significant: the lower the debt ratio, the higher the likehood that a firm can survive in the future, and so an increase in that ratio tends to be associated with a decrease in the distance to default. The operating margin gives an indication of a company’s profitability and, therefore, it is appropriate to positively link it with distance to default, based on the following observation: the higher a company’s profitability, the lower the probability of default. Indeed, operating margin appears to be significantly and positively related to distance to default. The retained earnings to total assets ratio helps to measure the extent to which a company relies on leverage. The lower this ratio, the higher its leverage, which again increases the risk of bankruptcy where the firm cannot timely fulfil its debt obligations.
However, in our model, the relationship is negative rather than positive and is not significant. The results are probably biased by the presence of the debt ratio, which is another indicator of leverage. Larger companies can be expected to be evaluated by the market as safer than smaller companies; surprisingly the association found is negative, suggesting that the market considers bigger companies riskier. Volatility is another fundamental indicator of creditworthiness. Merton’s structural credit risk model (1974) was the first to indicate that reduced firm value volatility also leads to lower risk premiums, and in the regression this relationship is indeed significant and negative. Lower volatility increases the value of the assets and leads to a rise in the distance to default. Finally, working capital to total assets indicates a company’s ability to pay back creditors in the short term. Those with a healthy and positive working capital should not have problems paying their bills, and should therefore have a larger distance to default. In line with this, the association observed is positive but not significant.
In order to evaluate the robustness of the results, two more panel least square regressions are run. This time, instead of using the natural logarithm of carbon emissions, carbon intensity is employed. Carbon intensity is the ratio between the level of emissions and total sales. This ratio is particularly used in the energy sector, where carbon emissions are compared against the megajoule of energy produced. Given the many different industries involved in the sample analysed, here carbon emissions are divided by sales. As before, first only carbon intensity is used as an independent variable, and then all the control variables are added.
The results are not different from the previous analysis. Carbon intensity is significantly and negatively associated with Merton’s distance to default. In addition, retained earnings/total assets and working capital/total assets are once again not significant, and size remains negatively related to distance to default.
An additional robustness check is carried out, as shown in model 5 in figure 2. Instead of using total disclosed emissions, only direct emissions are used. Since this level of detail is available only for a limited number of companies, the sample is reduced to 120 with 1,320 year observations. Therefore, a panel least squares regression is run using the same control variables and replacing the natural logarithm of total emissions with the natural logarithm of direct emissions.
The level of direct emissions continues to be significantly and negatively related to Merton’s distance to default but only at a 10% significance level. These weaker results could be due to the smaller size of the sample. In addition, in this case retained earnings/total assets is signficantly and positively related to distance to default, and working capital/total assets is significantly but negatively related. Surprisingly, the size variable is not significant.
Model 5 in figure 2 shows a regression with time fixed effects.The adjusted R-squared improves and our previous results hold: the relationship between the natural logarithm of emissions and distance to default continues to be negative and significant.
The Paris Agreement and the increased attention of investors to climate change issues imposes risks on companies with high CO2 emissions. Rigorous enforcement of existing environmental laws and the introduction of stricter criminal and civil penalties for polluters are expected for the future. This could result in a spike in costs and in impacts on issuers’ creditworthiness.
This paper investigated whether a firm’s CO2 emissions affect Merton’s distance to default. The results show that a higher level of emissions actually leads to a lower distance to default. Descriptive statistics already reveal the influence of CO2 emissions on the probability of default. The sample is divided into quintiles (and deciles) according to each firm’s level of emissions: we show that companies in the first decile or quintile (less polluting firms) have a higher distance to default compared to the most polluting firms. We find strong evidence that emissions are negatively associated with distance to default. These findings are confirmed using both the natural logarithm of emissions and carbon intensity. The baseline results hold, even excluding the energy and extractive industries. In unreported results, we additionally find that the carbon footprint decreases the distance to default following regulatory shocks such as the Paris Agreement, which reveal policymakers’ intention to implement stricter climate policies.
Given the outlook of increasing global temperatures, it is important to assess the impact of this on the macro-economy and financial markets. Rising temperatures may disrupt financial markets and the banking system. Our results show that firm creditworthiness is already affected by exposure to climate risks. Policymakers should carefully consider the impact of climate change risks on the stability of both lending intermediaries and corporate bond markets. The Task Force on Climate-related Financial Disclosures (TCFD) has developed voluntary, consistent climate-related financial risk disclosures for use by companies in providing information to investors, lenders, insurers, and other stakeholders. Our findings prove that the work and recommendations of the task force are justified as the amount of carbon emitted by companies provides investors with relevant information. However, transparency is only the first step. As carbon risks appear more pervasive and material for the global financial system than previously thought, the compelling issue for investors and financial regulators is how to manage or neutralise such risks once they have been identified and quantified.
References
Battiston, S., A. Mandel, I. Monasterolo, F. Schütze and G. Visentin (2017). A climate stress test of the financial system. Nature Climate Change 7(4): 283–288.
Capasso, G., G. Gianfrate and M. Spinelli (2020). Climate change and credit risk. Journal of Cleaner Production 266: 1–10.
Dyck, I.J., K. Lins, L. Roth and H. Wagner (2019). Do Institutional Investors Drive Corporate Social Responsibility? International Evidence. Journal of Financial Economics 131(3): 693–714.
Krueger, P., Z. Sautner and L. Starks (2020). The Importance of Climate Risks for Institutional Investors. The Review of Financial Studies 33(3): 1067–1111.
Merton, R. (1974). On the pricing of corporate debt: the risk structure of interest rates. The Journal of Finance 28(2): 449–470.