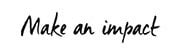
By Dominic O’Kane and Jean-Michel Maeso
In today’s world, investors need to be mindful of the impact of climate change on the price of the stocks they hold as these may be negatively impacted by extreme climate events (physical risk), and by regulatory or fiscal changes intended to enable the transition to a low carbon economy (transition risk). However, it is not immediately obvious how to find a climate risk measure that we can link to asset prices. Such a measure would be useful to quantify and hedge climate-related market risks.
In 2018, Engle et al. [2020] investigated the use of climate news as such a measure of climate risk, the idea being that the news media reports daily on all climate-related events, both physical and transition risk-related, and the more important the risk, the greater the market impact may be. They used the Wall Street Journal as its news source and a simple numerical measure of climate news obtained by comparing news articles to a corpus of climate-related words. They also used a black-box climate sentiment index generated by Crimson Hexagon from multiple news sources including social media. The authors then built hedge portfolios by regressing news index innovations on so-called climate characteristic portfolios and found that out-of-sample, using the WSJ climate news index (CNI) and Sustainalytics E-scores, the correlation between CNI innovations and hedging portfolio returns is 17%.
A theoretical contribution to our understanding of the relationship between green and brown[1] stocks, and the impact of unexpected information, is provided by Pástor et al. [2021]. The authors analyze the effects of sustainable investing using a two-factor equilibrium model, the factors being a market factor and an ESG factor. They argue that ESG preferences should move asset prices and that green stocks have negative alphas while brown stocks have positive CAPM alphas. The negative CAPM alphas are linked to investor tastes for green holdings and the use of these assets for hedging climate risk. A key conclusion that emerges from their approach is that green stocks can outperform brown stocks if there are unexpected increases in ESG concerns.
Ardia et al. [2022] tested the predictions of the model of Pástor et al. [2021]. Using US newspapers, they calculated a concern index based on the excess of negative over positive words weighted by risk concerns using the LIWC[2] lexicography. They found that on days with an unexpected increase in climate change concerns, green firm stock prices tend to increase while brown firm stock prices tend to decrease.
We extended this work by using a broader range of news sources and a more sophisticated set of climate news indices based on the latest natural language modelling approaches. We chose to use several leading and reputable English-language news sources, specifically the Financial Times, the Guardian, the New York Times, the Daily Telegraph and the LA Times. To the best of our knowledge, this study is unique in utilizing the Financial Times as a news source to construct climate news indices. However, using the Financial Times alone is not enough as we argue that the use of several news sources is crucial in capturing both the systemic and idiosyncratic components of climate news.
We then applied a range of language models to detect the importance of climate news. We first identified “climate change articles” as those containing either of the terms “climate change” or “global warming”. As we might expect, we find that the percentage of climate change articles published each month is closely linked to climate news events. This is shown in Figure 1 where we calculate the percentage of FT articles published by the FT and show that the peaks correspond to several significant climate change events – political summits and IPCC conferences and the election of President Trump.
Figure 1 : We measure the climate change attention (CCA) from 2005-2021 by calculating the percentage of daily FT articles
that contain the term ‘climate change’, the percentage that contain the term ‘global warming’, and the percentage containing either term.
We apply a 30-day moving average. The vertical lines are climate change-related events – see below for the corresponding numbered list of events.
Table 1: Identification of the most active climate news events seen in Figure 1.
For climate change conferences we have used the conference end date when the final agreement is usually announced.
We then constructed five different climate news indices (CNI) methodologies, one for each newspaper:
This resulted in 5 climate news indices, one for each newspaper and newspaper. We also computed a sixth aggregate index which took an average of the five other indices, appropriately normalized by the volatility of each newspaper’s climate index. To remove auto-correlation effects so we may correctly capture the unexpected changes in the climate news index, we modelled the climate news index as an AR(1) process and extracted the residual. This gave us the unexpected climate news innovations index (UCNI) time series.
We then measured the link between the daily UCNI for each climate news index approach and for each newspaper (and the aggregate) and the daily performance of portfolios of S&P 500 firms in which we buy green assets and sell brown assets over the period from 2012 to 2022. Note that to separate the portfolio into green and brown stocks, we used the combined Scope 1 and Scope 2 emissions taken from FactSet’s ISS ESG carbon emissions data.
We carried out several analyses. Our main finding is that there is a statistically significant link at the 1% level between the UCNI averaged over all the news sources, and the returns of a portfolio of brown-only S&P 500 firms. This result held for all the index construction approaches used but it did not work for individual news source indices. This leads us to two conclusions. First, a news index based on an average of news sources may be more powerful at capturing market-moving climate news events than one based on single newspapers. This can be explained by observing that a climate change news event that is reported by several newspapers at the same time is more likely to be systemic and market-moving than one that is reported by one or two newspapers. Second, brown assets are negatively exposed to climate risk (more risk and concern reduces their returns) as measured by climate news, while green assets have no statistically significant exposure to climate news. It suggests that the returns of climate risky assets may be skewed to the downside. This result does not appear to be in concordance with Pastor et al. and more work would be needed to determine why.
The research, from which this article is drawn, was produced as part of the research chair “Measuring and Managing Climate Risks in Investment Portfolios”, established with the support of Amundi.
A copy of the EDHEC-Risk Climate Impact Institute publication can be downloaded via the following link: The Impact of Climate Change News on Low-minus-High Carbon Intensity Portfolios.
A copy of the academic paper published in the Novel Risks issue of The Journal of Portfolio Management can be downloaded via the following link: "Investigating the Influence of News Sources and Language Models on Climate Beta Estimates".
Footnotes
[1] A "green stock" refers to a stock of a company that is involved in the green or a sustainable industry and can usually be identified by its low carbon emissions as a percentage of its revenues – this is known as its carbon intensity. A “brown stock” refers to the stock of a company that usually has a high carbon intensity.
[2] See https://www.liwc.app/.
References
Ardia, D., K. Bluteau, K. Boudt, and K. Inghelbrecht. 2022. “Climate Change Concerns and the Performance of Green Versus Brown Stocks.”, Management Science, forthcoming.
Devlin, J., M.-W. Chang, K. Lee, and K. Toutanova. 2018. “BERT: Pre-training of Deep Bidirectional Transformers for Language Understanding.”, ArXiv preprint arXiv:1810.04805.
Engle, R., S. Giglio, B. Kelly, H. Lee, and J. Stroebel. 2020. “Hedging Climate Change News.” Review of Financial Studies, Volume 33, Issue 3; pages 1184–1216..
Maeso, J.M. and O’Kane, D. “Investigating the Influence of News Sources and Language Models on Climate Beta Estimates”, The Journal of Portfolio Management, Volume 50, Number 2, December 2023.
Pástor, L., R. F. Stambaugh, and L. A. Taylor. 2021. “Sustainable Investing in Equilibrium.”, Journal of Financial Economics, Volume 142, Issue 2, pages 550–571.
Webersinke, N., M. Kraus, J. Bingler, and M. Leippold. 2021. “ClimateBert: A Pretrained Language Model for Climate-Related Text.”, ArXiv preprint arXiv:2110.12010v3.