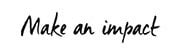
Industry Analysis
Factor-Based Approaches to the Design of Smart Bond Portfolios
Frank Fabozzi
Lionel Martellini
Riccardo Rebonato
The modern approach to factor investing (see for example Amenc, Goltz and Martellini (2013) for an application to the equity space) suggests that before applying a weighting scheme, we should first identify robust and economically-motivated sources of differential cross-sectional returns, which can be regarded as ‘factors’, with rational or behavioural interpretation, or ‘frictions’. In this context, more work is required both in academia and in the industry to start addressing these challenges in a careful way, before we are able to see the emergence of improved bond benchmarks that will provide investable answers to investors' needs.
An economic motivation is not just an academic add-on. Understanding the source and origin of the cross-section differential returns matters a lot from the point of view of a robust benchmark creation:
For the sake of brevity, we therefore refer in this article to all these sources of differential cross-sectional returns as ‘generalised factors’, but it should be kept in mind that, from the perspective of the creation and long-term profitability of investable factor benchmarks, the underlying differences can be very important. It turns out that the recent discovery about generalised factors that used to ‘work well’ for equities also seem to be effective in the fixed-income area does create an embarrassing explanation problem. As Asness, Moskowitz and Pedersen (2013) eloquently put it, “[t]he strong correlation structure among value and momentum strategies across such diverse asset classes is difficult to reconcile under existing behavioural theories, while the high Sharpe Ratios of a global […] diversified portfolio presents an even more daunting hurdle for rational-risk-based models.” So, we seem to find more and more factors and to observe that the ‘old’ factors seem to work even where they were not expected (or even supposed) to; however, we are farther and farther away from a unified understanding of why they do. As argued above, unfortunately this imperfect understanding does matter when it comes to building robust and stable investable portfolios.
Modern ‘generalised factor discovery’ in the fixed-income area is also made more difficult by the problem of proxies. Very often, a candidate factor (such as liquidity or value) is difficult to measure, or even to define, precisely. Furthermore, as recognised as early as 1993 by Fama and French (1993), a straightforward transposition of the factors that are most popular in the equity space (such as, for instance, value, momentum or low volatility) to the fixed-income arena is not straightforward. [3]
This has led to the proliferation of proxies, and, sometimes, to the creation of proxies of proxies. For instance, Asness, Moskowitz and Pedersen (2013) investigate value and momentum in fixed income, and circumvent the problem of defining value for bonds (a concept that, according to Fama and French (1993) ‘has no obvious meaning for […] bonds’ – see footnote 5) by arguing that “individual stock portfolios formed from the negative of past 5-year returns are highly correlated with those formed on BE/ME ratios in our sample. […] Hence, using past 5-year returns to measure value seems reasonable. …” The logic here is to use a proxy (the 5-year returns) to stand in for another proxy (value) for an unspecified latent factor. The choice may well be reasonable, but the link to the desired proxy (value), let alone to the latent factor, is neither transparent nor unique: indeed, in the literature a number of additional measures of value for bonds have been proposed, such as, the 5-year yield change in nominal yields, the difference in the 10-year yield to the 5-year inflation forecast (for real bonds), the 10-year yield spread to the short rate, not to mention a composite average of all three measures.
In addition, this concern is of relevance for the construction of robust benchmarks. As we have seen, despite the suggestive labels attached to the factors, what has actually been studied in the recent literature are often proxies more or less loosely associated to the additional fundamental quantities they 'stand in for'. This can create not only ambiguity, but also ample scope for data snooping and over-fitting. [4] Needless to say, the cost of over-fitting in-sample is poor performance out-of-sample.
Apart from the dangers of over-fitting and data mining, discovering more and more factor proxies (just in the equity space, Fama recently counted more than 350!) is not necessarily desirable from the point of view of the construction of a truly diversified portfolio. [5] Indeed, as Cochrane (2010) reports, “in 2007 and 2008, hedge funds found to their dismay that portfolios they had constructed to exploit multiple “signals” all fell at the same time”. This is exactly suggestive of a single source of “risk” corresponding to multiple signals of “return”. A multitude of putative proxies can therefore engender a false sense of high portfolio diversifiability, and obscure the fact that a very small number of underlying latent economic factors may manifest themselves through a variety of highly correlated measurable proxies. After all, if a factor proxy is associated with a true risk premium, the attending ‘excess’ return is simply a compensation for receiving good or bad pay-offs in periods of high or low consumption, respectively. High or low consumption, in turn, can be parsed in terms of a relatively small number of (often highly correlated) economic configurations, such as low growth, or high unemployment. A principled and parsimonious approach to proxy analysis is therefore essential, especially in the nascent field of fixed-income ‘smart beta’, but has arguably not received sufficient attention.
All these qualifications should be clearly kept in mind as we review in what follows the generalised fixed-income factors that have been identified most consistently in the modern literature. Among the best documented fixed-income generalised factors, one finds the following:
1. Low risk has been found both in corporate bonds and in Treasuries – see, for example, de Carvalho et al. (2014), Ilmanen et al. (2004), Frazzini and Pedersen (2014) and Howeling and van Zundert (2017). In the case of Treasuries, it is well known (Naik et al., 2016) from time-series studies that low maturity bonds offer a higher Sharpe ratio in virtually every economic environment. While these results are statistically very robust, their economic significance and portfolio exploitability is not obvious. This is especially true for Treasuries and high-yield corporate bonds, given that the recent very-low-yield environment has made the attainment of equity-like returns only obtainable with unfeasibly high leverage – de Carvalho et al. (2014) document a required leverage of 50 for the lowest-risk (and highest Sharpe ratio!) bonds. The existence of leverage-constrained investors also suggests a (regulatory/institutional) reason for the low risk generalised factor.
2. Momentum has been documented in a variety of asset classes, including fixed-income – as can be seen from the very title of the well-known paper of Asness, Moskowitz and Pedersen (2013), “Value and Momentum Everywhere”. It should be remembered, however, that momentum is not truly a generalised factor (i.e. an attribute of a security – such as its volatility – or of an issuer – such as the debt-to-equity ratio), but the result of a trading strategy. The origin of this well-documented feature is still hotly debated in academic circles. When it comes to fixed-income instruments, the profitability of momentum is also still debated. For investment-grade bonds, Khang and King (2004) and Gebhardt, Hvidkjaer and Swaminathan (2005) find reversal, while Jostova et al. (2013) find no momentum. In preliminary work, Rebonato (2017) uses the Treasury-derivate discount bond data by Gurkaynak, Sack and Wright (2007) to create self-financing (zero-cash) portfolios that are long ‘winners’ and short ‘losers’. With this strategy, it is found that momentum in US Treasuries is profitable over a relatively wide combination of training and investment periods. [6] Popisil and Zhang (2010) and Jostova et al. (2013) find momentum in the corporate high-yield market.
3. Value was identified as a factor for corporate bonds by L’Hoir and Boulhabel (2010) and by Asness, Moskowitz and Pedersen (2013) for US Treasuries. Above, we mentioned the difficulties in ascribing a precise meaning to ‘value’ in the fixed-income context, and the particularly ‘creative’ adoption of proxies and second-order proxies that this difficulty has generated. To solve these problems, current work (Rebonato and Hong, 2017) attempts to make use of affine structural models or of time-series return-predicting factors (see Cochrane and Piazzesi, 2005;
Cielsak and Povala, 2010), to establish cross-sectional differentiations in the returns of Treasury bonds. While initial results are encouraging, these early studies still require further analysis and confirmation.
4. Liquidity is a particularly promising rewarded ‘true’ factor in the fixed-income space, as poorer returns due to a reduction in liquidity are often associated with adverse economic conditions (and hence with periods of low consumption). Recently Acharya and Pedersen (2005) have put liquidity on a firm asset-pricing footing by embedding its treatment in a CAPM-like framework, and identifying three liquidity-related ‘betas’ in addition to the traditional market beta. [7] The problem with their approach is that liquidity is a latent quantity, and the choice of a suitable proxy is fraught with difficulties – see Adler (2012) for a discussion of the different aspects and ‘types of’ of liquidity. [8] Indeed, away from the equity arena, direct measures of liquidity gleaned from transaction-level data (such as bid-offer spreads) are both difficult to obtain, and of dubious reliability. [9] It is probably for this reason that very few studies document the link between excess returns and liquidity in the fixed-income area. In an attempt to remedy this situation, current work by Rebonato and Hong (2017) focuses on the establishment of a robust liquidity proxy for fixed income, constructed from publicly available price-based time series by modifying a methodology pioneered by Ludvigson and Ng (2009). They show that this measure of liquidity is closely linked to measures such as ‘liquidity and noise’ (Hu, Pan and Wang, 2013), and funding liquidity (Adrian, Etula and Muir, 2013), and argue that a large part of the yield premium provided by TIPS over US Nominal Treasuries can be explained as a compensation for liquidity risk factor. Rebonato and Naik (2016) also document the impact of liquidity on the pricing of TIPS relative to nominal Treasury bonds by using a variety of visible market proxies, but they do so without trying to combine them as in Rebonato and Hong (2017).
5. Carry can in general be defined as the funding of a riskier, longer-dated, higher-yielding strategy by shorting a lower-risk, shorter-dated and lower-yielding security. As in the case of liquidity, “carry” is likely to be linked to a true factor, in the sense that it rewards undiversifiable risk-taking likely to materialise in situations of market distress. This interpretation is indirectly confirmed by Lettau, Maggiori and Weber (2014), who apply the ideas behind the Downside-CAPM Asset pricing model, and show convincingly i) that the extra return afforded by a variety of carry strategies can be explained as compensation for poor pay-offs in periods of poor equity returns, and ii) that the well-known poor power of the CAPM model when it comes to explaining cross-sectional returns can be substantially increased once downside risk is taken into account. In the case of corporate bonds, carry is, of course, earned by investing in riskier, longer-dated securities while funding the long position in safer, shorter-dated bonds (perhaps Treasuries). By using yield-times-duration[10]as a measure of risk, de Carvalho et al. (2014) argue that, while longer-dated, lower-rated bonds offer the highest returns, low-risk, short-maturity bonds provide the best Sharpe ratios. Along similar, but not identical, lines, industry studies suggest that the best trade-off between extra yield and risk (the best Sharpe ratio) is offered just below the investment-grade rating.
6. Size as a generalised factor in fixed income has been recently analysed by Howeling and van Zundert (2017). They do so by looking at the total size of the company’s debt, rather than the size of the individual issue. In their empirical analysis, every month they form a portfolio made up of the 10% of bonds with the smallest company index weights. Of course, since smaller companies tend to issue smaller bonds, and since smaller issue tend to be less liquid, the size-generalised factor inevitably picks up a liquidity contribution. They find size to contribute to portfolio profitability when combined with Low Risk, Value and Momentum. They find that the returns from this multi-factor portfolio are up to three times higher than the market, and that the excess return cannot be explained by risk or by equivalent equity factors. We should keep in mind the caveats expressed above about the possible convergence of different factors in situations of market distress.
7. Fallen angels, defined as corporate bonds downgraded to just below investment grade, seem to offer a premium when investing for a short period (up to 6 months). The effect has been analysed by Staal et al. (2015) for a comparison of the profitability of an unconditional strategy that always long the Barclays US high-yield index with a fallen-angel strategy. [11]The same study reports a ratio of 4 to 3 in favour of the fallen-angel strategy for the relative Sharpe Ratios for the period from April 1998 to March 2015. Institutional frictions are the most likely explanation for the fallen-angel effect, which is often exploited by deploying downgrade-tolerant strategies, because a large number of mandates prohibit investment in non-investment-grade securities, and force liquidation after a downgrade.
New Frontiers in Smart Bond Portfolios
Overall, it seems that more research is clearly needed before risk premia can be as efficiently extracted in the fixed-income space as in the equity space, and this must be done with emphasis on the implementation constraints and data qualities issues, some of which are highly specific to bond markets. Now that the construction of smart beta equity portfolios has almost become a well-established technology, we see these challenges as an important new frontier in smart beta investing.
This article is one of many articles in Investment Management Review (IMR) special edition Autumn 2017 which covers important recent insights into the asset management industry. To access the full edition please click on http://www.imrmagazine.com/edhec-special-edition.php.
[1]Cochrane (2010) stresses the recent shift in emphasis in asset pricing research from variations in expectations to variation in discount rates.
[2]This clear distinction between ‘irrationality’-based and institutional-based source of differential cross-sectional returns can easily become blurred: the availability of the speculative capital that should arbitrage away irrationalities may, for instance, have become greatly reduced because of regulatory initiatives such as the Volker rule in the States, or the Liikanen proposal in the EUR area.
[3] ‘… explanatory variables like size and book-to-market equity have no obvious meaning for government and corporate bonds…’, page 4.
[4]On this point, for direct a discussion of investment implications see Abu-Mostafa, Magdon-Ismail and Lin (2012) and and Bailey et al. (2014).
[5] Bender, Briand, Nielsen and Stefek (2010) and Ilmanen and Kizer (2012) emphasise the diversification benefits of factor-based portfolio building. Their point is well-taken, as long as the underlying commonality of many factors, and the attending reduction in effective diversifiability, are recognised.
[6]The training period is defined the period over which the relative out- or under-performance of bonds of different maturities is monitored and used to construct the long/short portfolio. The investment period is the period over which the zero-cash portfolio is held.
[7] In addition to a (liquidity-corrected) market beta, additional terms appear in the pricing equation that are linked to the covariances between the asset illiquidity and the market return, the asset return and the market illiquidity, and the asset illiquidity and the market illiquidity.
[8] The recent literature differentiates between market liquidity (linked to the ability to transact in market size without significantly affecting the price), and funding liquidity (linked to the availability of capital to fund positions in risky assets.)
[9]For instance, as liquidity begins to deteriorate, dealers tend to reduce to a ‘market size’ in which they are willing to transact before changing the bid-offer spread.
[10]For a justification of Duration-Times-Yield as a measure of bond risk, see Fisher (2006).
[11]See Exhibits 17 to 19 in the above-mentioned paper for more detail.
References