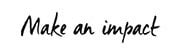
by Riccardo Rebonato, Professor of Finance, EDHEC-Risk Institute, EDHEC Business School
Feature
In the wake of the 2007–2009 financial crisis a broad consensus has been reached that relying on purely statistical (usually frequentist) techniques to manage financial risk is both unwise and difficult to justify. Stress testing has often been touted as the answer (or at least one of the answers) to the shortcomings of managing financial risk using purely statistical tools. Regulators have given additional reasons to ‘treat stress testing seriously’ through the association of how much capital systemically important financial institutions (SIFIs) should hold and the outcomes of stress-testing exercises.[2] As a result of this, there has been a resurgence of academic interest in the area of stress testing, including a recent contribution from Rebonato (2018):
The first two contributions are particularly relevant for (micro-) prudential applications of stress testing and the latter for portfolio allocation. Here we summarise the three main contributions of the paper, explain why they are important, and briefly describe how the proposed approach fits in with current research on stress testing. The readers are referred to Rebonato (2019) for further details.
A good starting point is the useful distinction made by the ECB (2006) between historical, hypothetical, probabilistic and reverse-engineered scenarios. Broadly speaking, historical scenarios apply past moves in market and/or macrofinancial variables to today’s portfolio: the post-Lehman events, the bursting of the dot.com bubble or the LTCM crisis are typical examples.
Hypothetical scenarios apply to the current portfolio moves in market and/or macrofinancial variables that are expected to occur if some subjectively chosen scenario were to materialise: the breakup of the EURO currency block, or the outcome of a referendum such as Brexit fall into this category.
Probabilistic scenarios apply shocks obtained from a joint distribution of market and/or macrofinancial variables that has been estimated on the basis of historical data; they may (but not necessarily) employ statistical techniques — such as Extreme Value Theory — designed to probe the tails of the distribution.
Finally, reverse-engineered scenarios try to identify the most likely moves in market and/or macrofinancial variables that can give rise to a given maximum acceptable loss (see for example Rebonato, 2018 for a recent discussion of this topic).
The distinctions are more blurred than these concise definitions may suggest: ‘objective’ probabilistic studies still require expert knowledge-based subjective judgement (such as deciding which data are relevant to current market conditions, and which trade-off should be struck between relevance and abundance of data); and even the most subjective hypothetical scenarios are necessarily informed by statistical information about past occurrences of ‘similar’ or ‘relevant’ stress events. Indeed, it has been forcefully argued that the current unsatisfactory state of stress testing calls for a stronger role of judgement in probabilistic approaches, either in output (see for example Alfaro and Drehmann, 2009) or inputs (as in Breuer, Jandačka, Mencia and Summer, 2009).
Whatever approach is used, the Basel Committee on Banking Supervision (2005) recommends scenarios that are at once plausible, severe and suggestive of risk-reducing action. It is important to stress that the notion of plausibility can be easily, but unhelpfully, confused with some level of probability mass for a given set of events obtained from a statistical approach (probabilistic scenario): because of its analytical tractability, this is indeed the most common approach, as in Breuer, Jandačka, Rheinberger and Summer (2009), Breuer, Jandačka, Mencia and Summer (2009)[3], Flood and Florenko (2013), Glasserman, Kang and Kang (2014). However, an event like the break-up of the Euro currency — with the attending unprecedented changes in dependencies among different variables and, perhaps, the creation of new variables, such as the ‘new drachma’ — was very plausible in the summer of 2012, but simply not present in the data, and therefore impossible to tackle with purely probabilistic models. Probabilistic and hypothetical scenarios therefore complement each other.
Another challenge with stress analysis often mentioned in the literature is what is referred to in Rebonato (2018) as the dimensionality problem: typically, a relatively small number of macro or market variables are shocked (probabilistically or subjectively), but the value of a realistic portfolio depends on granular variables that are often orders of magnitude more numerous. Breuer, Jandačka, Rheinberger and Summer (2009) show that the optimal way to propagate shocks from the few to the many variables is to set the non-directly shocked factors to their conditional expectation given the value of the stressed factors. The technique we present in Rebonato (2019) makes use of this insight in a novel and efficient way.
Stress testing has importance beyond macro- and microprudential concerns. Asset managers are keenly interested in coherently integrating the possibility of severe market distress into their asset allocation procedure. Since diversification is key to this process, and since codependencies between risk factors can dramatically change in periods of financial distress, this poses an obvious challenge. The challenge is compounded by the need, further discussed below, to coherently integrate ‘business-as-usual’ and distress portfolio allocations: arguably, as the probability of a distressed event goes to zero, the two allocations should converge. While this condition can be recovered in the case of probabilistic scenarios, it is not easy to ensure this in the case of hypothetical scenarios. (See Rebonato and Denev (2014) for applications of stress testing to portfolio management, and for one possible solution to this problem.) In this paper we offer a simpler and more financially justifiable solution.
These problems (and many more, not mentioned for the sake of brevity) have been widely recognised and amply discussed in the literature. Perhaps because of the greater emphasis placed on probabilistic approaches, much less attention has been paid to another important problem that one invariably encounters with hypothetical scenarios, but which arises in a latent form in probabilistic methods as well — a problem the solution to which is a key contribution of this paper. It can be stated as follows.
When prices of (usually a large number of) assets shift significantly and in ‘wild’ patterns, it is important to ask whether these price configurations are attainable given some basic financial requirements, such as the absence of large arbitrage opportunities. We know for instance from financial theory that a relatively small number of factors should and can satisfactorily describe the changes in the prices of all assets. These factors can include liquidity and market sentiment — factors that may be shocked very strongly, and perhaps to unprecedented extents and in never-before-realised configurations, in stress situations. Moves in all these factors are generally unconstrained and can be as ‘wild’ as one may wish.
However, the dimensionality reduction (modulo idiosyncratic terms) when moving from the space of the factors to the space of the asset prices imposes serious constraints on possible price moves. These financial consistency concerns are prominent in term structure modelling, where statistical (VAR-based) and model (no-arbitrage-based) approaches have been used for prediction purposes. Indeed, in the case of fixed-income asset pricing one of the recurring concerns is that the predictions produced by purely statistical models are ignorant of no-arbitrage conditions.[4] But there is nothing special about fixed-income securities: if we believe that prices should reflect security-independent compensations for assuming the undiversifiable factor risk, the issue of financial realizability of arbitrarily assigned price moves remains, particularly in the asset management context.
The potential ‘impossibility’ of arbitrary price moves is of course very apparent when dealing with hypothetical scenarios. One may think that this should not be the case with probabilistic scenarios, at least to the extent that the underlying joint distribution of price changes has been obtained from historical market data (data which at the time of collection must have embodied the financial constraints alluded to above). However, when dealing with severe scenarios, one is typically extrapolating into the tails of joint distributions where actual market occurrences are very rare or perhaps absent altogether. Frequentist techniques such as Extreme Value Theory are very well understood, but they "know nothing" about financial constraints. Rebonato (2018) shows how these financial consistency constraints can be naturally satisfied.[5]
In summary, these are the requirements that a useful and theoretically justifiable stress testing programme should satisfy:
Given this context and the requirements outlined above, this is what the approach in Rebonato (2018) can offer:
1. it firmly roots the stress-testing exercise in well-established financial (asset-pricing) theory. This makes it of particular interest to portfolio managers; however, its solid theoretical grounding constitutes a more generally desirable feature insofar as, no matter how severe the scenario considered, the outcome should still be compatible with fundamental conditions of no arbitrage. (See footnote 16 for more on this point.) When, instead, security prices are more or less arbitrarily shocked following intuition and ‘hunches’, there is no guarantee that the resulting prices should reflect any feasible set of ‘fair’ compensations for the exposures to the underlying risk factors — and, indeed, in naive approaches in general they will not;
2. it allows for an approximate estimation of the probability of an assigned scenario. As mentioned above, this is essential for capital adequacy purposes;
3. it naturally suggests corrective actions, thereby addressing the concerns of the Basel Committee on Banking Supervision (2005);[6]
4. it is built in such a way as to automatically satisfy what we call the consistency and continuity conditions: these reflect the requirements that, as the probability of the stress event goes to zero, the covariances among the risk factors should revert to their ‘business-as-usual’ values, and that they should do so without discontinuities. Again, this condition is essential for portfolio managers, who would naturally want, but usually fail, to see their ‘normal-times’ optimal asset allocations recovered as a limiting case when the probability of a given stress scenario goes to zero;
5. it provides a way to consistently propagate the stress event from the prices of risk factors moved ‘by hand’ to the potentially very large number of securities in a complex portfolio, and it does so in such a way as to satisfy the requirements set out in Breuer, Jandačka, Rheinberger and Summer (2009). Doing so allows one to handle the dimensionality curse alluded to above;
6. once a considerable amount of background preparation work is carried out once and for all, the stress-testing procedure we recommend can then be conducted almost in real time. This addresses IMF-like concerns about real-time intervention.
It has been argued (Rebonato, 2010; Rebonato and Denev, 2014) that a Bayesian-net approach is best suited to producing coherent scenarios. The practical and conceptual advantages offered by this technique over competing approaches are i) the ease of interpretation, ii) the ability to carry out of sensitivity analysis and iii) the critical interrogation by intelligent but not necessarily mathematically-versed decision makers — and, of course, iv) its solid conceptual and mathematical basis. For this reason, to ensure the following discussion in Rebonato (2018) is concrete, it is cast in the language of Bayesian nets. However, the solutions offered are applicable to any stress-testing approach in which the shocks to a number of macro factors and the associated probabilities can be assigned, and must be propagated to the portfolio prices.
Needless to say, assigning the joint probabilities of these complex shocks in a logically coherent manner is not easy, and this is why Rebonato (2018) recommends the use of Bayesian nets. However, we remain agnostic in the treatment that follows as to how the shocks and joint probabilities have been obtained.
Again, the reader is referred to Rebonato (2018) for implementation details, but these are the main results:
We strongly believe that these results make an important step towards using stress testing in a robust, theoretically justifiable, effective and intuitively appealing way.
Footnotes
[1] Rebonato R, (2019), A financially justifiable and practically implementable approach to coherent stress testing, Quantitative Finance, 827-842.
[2] For a survey of the stress testing-related regulatory initiatives of the post-Basel-II period, see Cannata and Quagliariello (2011), Chapter 6 and Part III in particular.
[3] Breuer, Jandačka, Mencia and Summer (2009) clearly highlight this aspect of model risk — also raised in Berkowitz (2000) — for their approach, which is based on the Mahalanobis distance: "We measure plausibility of scenarios by its [sic] Mahalanobis distance from the expectation. This measure of distance involves a fixed distribution of risk factors, which is estimated from historical data." (page 333).
[4] See Diebold and Rudebush (2013) for a discussion of the topic, and Joslin, Anh and Singleton (2013) for the conditions under which non-statistical information improves the predicting power of probabilistic models.
[5] Purely historical scenarios are immune to this criticism. Useful as they are, their shortcomings are well known.
[6] The stress-testing approach we propose is built on causal foundations. For a thorough discussion of how a causal (as opposed to associative, as embodied in statistical correlations) organisation of information leads much more directly to intervention, see Pearl (2009) and Pearl and Mackenzie (2018), Chapter 7 in particular.