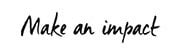
By Dr Bernd Scherer, Research Associate EDHEC, Head of Quantitative Portfolio Management, ADIA and Milot Hasaj, Senior Quantitative Analyst, Hauck-Aufhäuser-Lampe Asset Management
This article by Dr Bernd Scherer and Milot Hasaj has been originally published in the October newsletter of the Institute. To subscribe to this complimentary newsletter, please contact: [email protected].
Context
The European Union Sustainable Finance Disclosure Regulation (SFDR)[1] aims to increase transparency on the integration of sustainability risks and the consideration of sustainability impacts by the financial services sector with a view to helping investors to make informed choices and steering private capital towards investments with progressive sustainability contributions. Under SFDR, asset managers (and other financial market participants) are required to explain how they integrate environmental, social and governance (ESG) considerations into their investment processes[2] and to provide sustainability-related information with respect to financial products. Three types of sustainability disclosures are required for financial products: sustainability risks (financial materiality lens), principal adverse impacts (impact materiality lens), and proof of sustainability claims. In the latter respect, products that either “promote” environmental or social characteristics or have “sustainable investment“ as their objective are subjected to additional disclosures, as per SFDR Article 8 and Article 9, respectively.
Since 2019, Article 8 and 9 equity funds have received over three times more cumulative inflows than their non-ESG counterparts (sometimes termed Article 6 funds in relation to the SFDR provision imposing transparency of the integration of sustainability risks) – the SFDR is certainly a success in terms of driving flows towards newly established ESG funds and driving funds to reset and adopt the consider ESG characteristics beyond the lens of financial risks and opportunities.
In an article published in the Journal of Asset Management (Greenlabelling: How valuable is the SFDR Art 9 label?, August 2023), we look at whether categorisation under SFDR Article 9 induces more significant inflows than Article 8 funds. This question is highly relevant for regulators and asset management firms alike. Regulator want to steer investment flows into funds with environmental and social impact. However, Berk and Binsbergen (2021) argue that the impact on the cost of capital of impact investing – measured to be a mere reduction of 0.35% in capital costs – has been too small to meaningfully affect real investment decisions. According to this view, more flows are needed to impact the capital costs of progressive and regressive firms in terms of sustainability. If investors treat SFDR categories as labels, the regulator's task becomes far easier. It must then enforce a labelling regime to lower capital costs and make environmentally positive investments NPV-positive. For asset management firms, it is vital to determine the marginal AUM flows that come with heightened regulatory and reputational risks from creating Article 9 funds. This brings us back to our main research question. Does the Article 9 ‘label’ increase flows, or does it only help win the proverbial fruit basket or thought leadership coin? We must establish causality and move beyond a naive correlation analysis to answer this question.
Our paper also fits into the broader context of empirical research that is less obsessed with new theoretical models but instead relies on novel methods or datasets to show causality.
Researchers often use Directed Acyclical Graphs (DAGs) to spell out their identifying assumptions. They help them to pin down what causal effect they can identify and what makes good or bad control variables. Alternatively, we can look for natural experiments in the data to establish causality.
We view the 2022 SFDR category changes from Article 9 to Article 8 triggered by regulatory uncertainty as a quasi-experiment that allows us to study the impact of SFDR classifications on flows. To analyse the impact of category change as a form of exogenous intervention, we adopt the difference-in-differences method in line with academic research on related questions.[3]
Methodology
We need to identify the effect of category ‘downgrade’ (treatment or intervention) on flows (outcome). To isolate the impact of downgrades from other influences that might have happened simultaneously, we use another group that never experienced a downgrade and call this group the control group. Ideally, the control group closely resembles the characteristics of the treatment group. The critical assumption is that trends in the control group are a good proxy for what would have happened to the treatment group if the treatment had never happened. In other words: we use the control group to build a counterfactual for the absence of SFDR category changes. More precisely, we look for how much more flows of the downgraded funds changed relative to flows for non-downgraded (but otherwise comparable funds) when we move from before to after treatment. Mathematically, we compute this difference in differences from a two-way fixed-effect regression (TWFE).
Here i and t are individual/group and year subscript and variables and
denote individual and time-fixed effects. The average treatment effect of the treated (ATT) is the estimated coefficient b associated with the variable
, our treatment variable. It takes on a value of one for those funds that received a change in the SFDR category from Article 9 to Article 8 in the period that followed the downgrade.[4] All other entries are zero. Flows are denoted by
. Suppose our sample contains n funds that experienced a downgrade in their SFDR category (treatment group) and m comparable funds that did not (control group). Our variable
then has 2(n+m) data points, i.e., one data point for each fund in both the control and treatment period.
What assumptions are needed to interpret as the causal effect of changes in SFDR category on fund flows? Most importantly, we require exogeneity, i.e.,
and
need to be independent. This means the absence of other coincident changes which could have affected flows differently in treatment and control period. It also means the lack of anticipatory effects (flows did not already change in the first period due to the anticipation of category changes). We believe both conditions are satisfied as the category changes surprised investors and have been implemented at different times for firms. Using a cross-section of asset management firms makes it increasingly unlikely to assume that category changes coincide with confounding external events.[5] All systematic differences between funds (marketing efforts) are accounted for, provided they are constant across time. TWFE regressions identify the ATT in a two-period setting, i.e., where all units that receive treatment are treated simultaneously.
Table 1 summarises some of our results. For a large majority of asset management firms, the is negative. Reclassification reduces fund flows between -4.1% and -32%. Exceptions are Invesco, BNP and HSBC. None of our estimates is statistically significant. All fund families show 95% confidence bands that do cover zero.
Table 1: ATT From Two-Way Fixed Effect Model
ATT estimate from two-way fixed effect model for individual firms. Reclassification dates differ between DEKA (30th of August) and the remaining firms (30th of November 2022).
There are two problems with our analysis so far. First, we estimate the impact of fund reclassifications for each fund family separately. Second, our estimate for b captures the average impact across the entire post-announcement period, but fund groups changed their categories at different times. In order to relax these assumptions, we use recent advances in econometrics and employ the Callaway and St. Anna (2021) model and its R implementation to estimate the ATT in event-time to capture this effect. We use all data simultaneously and our model distinguishes between two fund groups. Funds that have been reclassified at the end of August 2022 (all Deka Art 9 funds) and those, that have been reclassified later, i.e., at the end of November 2022 (the remaining fund families). Table 2 shows the results. We find a substantial and statistically significant effect of, on average -10.27% in the immediate month following reclassification. All other estimates are insignificant.
Table 2: ATT across Event Time from CSA Model
CSA model with staggered intervention time (31st of August for DEKA and 30th of November for all other firms)
Reclassification makes investors leave previous Article 9 funds. After this adjustment, there is no difference in flows between old and new Article 8 funds (i.e., those recently reclassified from Article 9).
Summary
We use the recent reclassification of Article 9 funds as a natural instrument for identifying the importance of SFDR categories on fund flows and find statistical evidence that Article 9 funds attract more significant flows. We view this result as good news for regulators and asset managers alike. Regulators will become more confident they can channel flows into funds that have sustainable investment as their objective, and asset managers will have a continued business case for Article 9 funds.
Footnotes
[1] Regulation (EU) 2019/2088 of the European Parliament and of the Council of 27 November 2019 on sustainability‐related disclosures in the financial services sector.
[2] Disclosures for in-scope financial market participants and advisers pertain to policies in respect of sustainability risks (Article 3), adverse sustainability impacts (Article 4) and remuneration in relation to the integration of sustainability risks (Article 5). Additional disclosures (Article 10) apply if the participant offers a product that “promotes” environmental or social characteristics (Article 8 product) or has sustainable investment as its objective (Article 9 product).
[3] For example, Albuquerque et al. (2020) use difference in difference estimation to capture the link between the performance of firms with higher Environmental and Social ratings and the COVID-19 pandemic.
[4] Angrist and Pischke (2009) provide an introduction into the econometrics of the difference of difference estimator.
[5] The only potential exception are marketing efforts that might have cut back after the category change and hence affected flows. However, we cannot observe this variable.
References
Angrist J. D. and J. S. Pischke (2009). Mostly Harmless Econometrics: An Empiricist's Companion, Princeton University Press
Albuquerque, R., Koskinen, Y., Yuang, S. and C. Zhang. (2020). The Resiliency of Environmental and Social Stocks: An Analysis of the Exogenous COVID-19 Market Crash. Review of Corporate Finance Studies, Oxford University Press, vol. 9(3), pages 593-621.
Berk, Jonathan B. and van Binsbergen, Jules H., The Impact of Impact Investing (August 21, 2021). Stanford University Graduate School of Business Research Paper, Law & Economics Center at George Mason University Scalia Law School Research Paper Series No. 22-008,
Callaway, B. and Sant'Anna, P. H. (2021). 'Difference-in-differences with multiple time periods, Journal of Econometrics 225(2), 200–230.