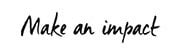
Industry Analysis
Improve digital MiFID profiling using behavioral smarts
Jurgen Vandenbroucke, PhD, Research Associate, EDHEC-Risk Institute, Head of Innovation at KBC Asset Management NV, Belgium and Lecturer in Financial Engineering at University of Antwerp, Belgium
(source: https://etendering.ted.europa.eu/cft/cft-display.html?cftId=3823)
Jurgen Vandenbroucke is contributing to the aforementioned study with a section on investor profiling. The draft version of his contribution is reported below. Any opinions are his own, and do not necessarily match the opinion of institutions Jurgen Vandenbroucke is affiliated with.
Investor profiling
For investor profiling purposes it is standard practice that investors are appointed to the profile that best fits their balance between risk and reward, based on a number of standardized questions.
This approach suffers from at least two shortcomings.
First, as stated by Nobel laureate Daniel Kahneman (2009), “people are poor forecasters of their future emotions and future tastes”. Questionnaires are unlikely to be good predictors of actual future behavior. Second, investor profiles restrict to an assessment of the client’s willingness to bear risk in order to obtain higher returns. Behavioral finance convincingly argues that actual human behavior is more refined.
This section outlines two ways to improve investor profiling:
The end result is a multi-dimensional investor profile, obtained through interaction and revealed preferences.
Improve the content – WHAT?
The European Securities and Markets Authority (ESMA) in a recent consultation paper on MiFID2 suitability requirements calls for an “assessment of suitability in the light of behavioral finance findings.” (ESMA, 2017, p.9).
Current profiling procedures hinge on the balance between long term expected return on the one hand and uncertainty about future realized performance on the other hand. Higher return expectations come with a higher uncertainty about actual future performance. This basic trade-off is one of the fundamentals in classical finance and makes perfect sense if one thinks about it slowly. Yet, at times, financial market conditions change quickly and trigger fast, intuitive or instinctive reactions; some of which challenge the logical long term trade-off.
Behavioral finance over the last decades has documented how actual human behavior can be better understood. ESMA now calls to include behavioral finance findings in the profiling of investors as it improves suitability of investment advice and contributes to investor protection.
Improvement of investor profiling based on behavioral finance findings can be achieved in alternative ways. Important to note is that such improvement need not be in conflict with the current approach. In fact, the best-selling title “Thinking, fast and slow” of Nobel laureate Daniel Kahneman illustrates the complementarity of a classical and behavioral approach. It is a targeted combination of both that ensures improvement.
The outcome is a two-dimensional investor profile that combines classical and behavioral investor preferences.
The first dimension of such enhanced investor profile covers the classical balance between long term expected return versus the predictability of realized performance. This is the focus of existing standard investor profiling. When considering to invest, the decision maker is assumed to make a projection of likely future outcomes, and balances the average, i.e. the measure for reward, versus dispersion, i.e. the measure for risk. Figure 1a provides a schematic representation. The decision to invest has to be made now, at the outer left of the chart, not knowing what the future will bring. Each erratic line is a projection of how the future may unfold. The appreciation of the investment is guided by balancing at any given investment horizon, represented by the black arrow, the average and dispersion of the likely outcomes. People may differ in how they balance risk and reward, hence the different classical risk preferences. In general however, thinking about such trade-off slowly, “people prefer more to less and dislike risk”. When choosing between two investments with the same reward, most people prefer the one with the lowest risk. When choosing between two investments with the same risk, most people prefer the one with the highest reward.
The second dimension of an enhanced investor profile deals with behavioral elements. How do people behave once invested? Figure 1b repeats the same visual representation but invites to read from left to right as if you travel through time. This is effectively what happens once invested. The portfolio value will evolve along the waves of financial markets, with ups and downs. From a classical, rational point of view, people are supposed to treat ups and downs equally and keep their focus on the long term trade-off between risk and reward. However, behavioral finance convincingly shows that for most people losses loom larger than gains. Also in everyday life people tend to take more effort to prevent a loss than to pursue a gain. In the context of investing many people react to changing market conditions in a way that challenges rationality. Their intuitive, fast reaction is largely explained by so-called loss aversion but, again, people may differ in how they balance interim gains and losses.
An investor profile that is supposed to stand the test of time includes at best both aspects of human behavior. Both aspects are complementary. It is perfectly possible that two investors with the same classical investor profile nevertheless differ in their concern of losing money. Like with any positioning system it takes two coordinates to locate oneself in the world of investing. See Vandenbroucke and Fortuna (2019) for a tested implementation.
Improve the method – HOW?
Although compliant with regulation, investor profiling based on questionnaires provides poor guidance of actual future behavior.
Researchers of the Dutch financial regulator AFM recently reported on a comparison of alternative quantitative methods to assess investor preferences, see Meeren et al. (2019). One of such methods was used in Chapman et al. (2018) to map behavioral preferences of U.S investors, i.e. the second dimension of the investor profile from the previous subsection.
Financial institutions have nevertheless focussed on phrasing the questionnaires as effectively as possible because for long an alternative was lacking. Today, the age of digitization offers new possibilities. Quantitative methods are much more effective since they are dynamic and personalized. While very hard to implement in a traditional setting, a digital application is straightforward.
The reader may consult the references to obtain deeper knowledge on alternative quantitative methods. The preferred method will likely differ depending on whether the assessment deals with the long term trade-off between risk and reward, or with the reaction to interim gains and losses. The general approach however, is to present the investor a personalized sequence of choices of the type shown in figure 2.
The sequence is personalized because the terms in the current setting of the problem depend on the revealed preference in the previous setting. The sequence stops if a convergence criterion is met, which irrevocably unveils the investor’s point of indifference between long term risk and reward or interim gains and losses. While stylized in figure 2, the principle can easily be gamified in a digital setting and preserve its superiority over a static, generalized questionnaire.
Preferred solution
Supporting retail investors in making more informed investment decisions starts with an accurate mapping of investor preferences.
A future-proof profiling procedure improves the current standard approach in two ways:
In the future, ideally, the investor profile effectively belongs to the investor. Citizens carry their ID to identify themselves as a person. Investors should similarly carry their investor profile to inform on their investor preferences. The investor profile should therefore be generic and interpretable by “all” financial institutions. Financial institutions may differ on the solution or advice they link to particular investor preferences, but the investor profile as such ideally belongs to the investor and is hence transferrable. Investors should not have as many profiles as there are financial institutions.
References
Chapman, J., Snowberg, E., Wang, S. and C. Camerer (2018), “Loss attitude in the U.S. population: Evidence from dynamically optimized sequential experimentation (DOSE)”, National Bureau of Economic Research, Working Paper 25072.
European Securities and Markets Authority (2017), “Guidelines on certain aspects of the MiFIDII suitability requirements”, Consultation Paper ESMA35-43-748.
Kahneman, D. (2009), “The myth of risk attitudes”, Journal of Portfolio Management, p.1.
Kahneman, D. (2011), Thinking, fast and slow, Allen Lane.
Meeren, G. van der, De Cloe-Vos, H. and A. Van Geen (2019), “Meet risicobereidheid met een kwantitatieve methode”, Economische Statistische Berichten.
Vandenbroucke, J. and G. Fortuna (2019), “Loss aversion implied by a risk-based questionnaire”, Journal of Wealth Management, p. 39-48.