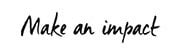
Industry Analysis
Machine Learning for Investment Decisions: A Brief Guided Tour
Professor John M. Mulvey, Bendheim Center for Finance, Center for Statistics and Machine Learning, Princeton University
1. What’s happening in machine learning?
The area of machine learning has generated much excitement and some trepidation due to surprising recent breakthroughs. Many Tech and FinTech firms are betting that the pace of AI and machine learning will only accelerate and lead to massive efficiencies and improvement in business processes. What jobs will be largely replaced or enhanced or created? This is a primary question to be answered going forward.
From the standpoint of investment decision-making, there have been striking improvements in select area, such as high frequency hedge funds.But the majority of strategic investment decisions are not so far distant from technology available 5 or even 10 to 20 years ago.
The growth of machine learning is due primarily to the ready availability of data at the micro-level. By this, we refer to data that supports individuals and their everyday behavior and decisions. The techniques of data science have been evolving and improving over the past few years, but many of the advantages can be traced to micro-level data and techniques that deploy the data in innovative and robust ways.
Some prominent examples of micro-level data include the following:
Amazon Go stores where computers watch individuals regarding selecting products
Drones checking out parking lots at shopping centers
GPS systems for auto insurance
Lower life insurance costs for non-smokers, people with healthy lifestyles
In the service sector, the primary goal of applying AI/ML is to strive to achieve FEB = {faster, easier, better}. Thus, firms have built platforms that give customers ability to almost immediately purchase goods (and to some extent) services without much worry about the quality of the products, simple delivery and return steps, and provide vast information on previous user experiences.The process is so straightforward and extendible to other applications. An example is the approval or denial of loans for individuals by fully automated risk estimation systems. These platforms collect massive amounts of micro-level data.
2. What’s happening in investing?
Over the past decade, many institutional investors have shifted their assets to illiquid “alternative” securities, including real assets, private equity, leverage debt, and hedge funds [6].This shift began with prominent U.S. university endowments, especially Yale University, and has led to superior performance in many cases. Among the asset categories, private equity stands out for its reliable and excellent returns.Public markets have joined this trend with the introduction of products that allow investors access to some of these new structures and opportunities. Many of the newer securities and deals have relatively complex multiple risk factors.
3. Illustrative Applications
This section reviews a few curated areas that are ripe for applications on machine learning for improving investment decisions. The choice of applications is personal, reflecting our ongoing research projects. Many others have benefited by applying machine learning, including high frequency trading, market making, contact engagements, new products and service development, and targeted advertising. It is clear that data science is remaking large segments of the financial service economy.
a. Factor investing (discovering significant risk features)
Factor investing has become a popular area for institutional investors [1][2][5]. A key principle is to understand the drivers of risks for any security so as to improve diversification.Today, many securities embed multiple risk factors. An example is private debt. These securities are levered, illiquid, act as bonds during normal periods, and mimic equities during crash period due to defaults.Thereby, investors spend time analyzing the underlying risk factors via methods such as regression and related techniques.In machine learning, similar quests are undertaken to pinpoint, for example, features that can forecast outcomes such as health interventions, e.g. genes that might lead to curing cancer via immunotherapy.Factor investing is equivalent to feature investing.And the goal is the same: discovering robust and durable features/factors.Machine learning algorithms can improve the identification and loadings of the underlying factors.Exhibit 1 shows the performance of a several equity long-short factors. The relative performance of a number of factors, while excellent over the long period, has become lower since around 2005. References [3] provide details of some of these studies.
Exhibit 1
Performance of Popular Micro Risk Factors Compared with U.S. Equities
b. Network graphical analysis (unsupervised learning)
One of the primary domains in machine learning is “unsupervised learning.” As compared with supervised learning in which sample data is labeled with correct answers for classification purposes, the goal is to discover patterns in data. Again, the availability of micro-level data gives rise to special circumstances. To this end, many studies exist that link objects – words, individuals, firms – in a network graph. Nodes define the objects. The arcs in this network indicate relationships between the objects, and geographical closeness displays affinity. An example appears in Exhibit 2. Here the objects are a set of countries and the relationships identify closeness on the movement of currencies pairs over the 2015-18 period. There are a number of interesting relationships to visualize, such as the closeness of the Japanese Yen and the Swiss Francs, likely due to the flight to quality characteristics of these currencies during volatile periods.
Exhibit 2
Network Graph of a Group of Currencies (2015-2018)
c. Estimating risks
The availability of micro-level data for individuals is a natural starting point for estimating risks at the atomistic level. The area is called precision medicine in health care – pinpointing the probability of specific outcomes for procedures such as target interventions for a single individual. Similarly, in finance, studies have become available in the form of personalized financial planning systems. And large institutions such as insurance companies are aiming to identify risks accurately so that costs can be tailored to each individual. Take the case of a GPS enabled device in an automobile in order to monitor the driver’s speed and carefulness (or otherwise) and give discounts when appropriate. Likewise, life insurance companies have offered discounts for healthy behavior for many years – no smoking, increased daily walking, and these arrangements are becoming more accurate with micro-level data and ML algorithms.
The estimation game extends to the economy as a whole. To this end, a recession often begins with individuals who become stingy with spending and businesses who cut back on capital improvements at the same time. Monitoring individuals and firms closely, thereby improves the forecasting accuracy. Machine learning concepts are easily adapted to these agent-based exercises and will have an impact on investing decisions in the future.
d. Multi-period financial planning
Multi-period financial planning models can be applied to numerous practical investment problems. An example involves planning for retirement for individuals: What amount should be saved? How to invest capital? When to retire? Each of these issues can be addressed by means of a multi-period stochastic model.Unfortunately, these problems are notoriously difficult due to the curse of dimensionality and other issues such as constructing stochastic scenarios that give a reasonable depiction of future conditions. Machine learning concepts offer assistance to overcoming these barriers. A promising development is the use of deep neural networks (DNN) in multi-stage portfolio planning [8]. The DNN generates a massive number of intermediate parameters (millions) and connections and has been highly successful in zero-sum games (e.g. Chess, Shogi and Go) where an exponential number of paths exist. The DNN runs alongside other algorithms, including Monte Carlo Tree Search, for fast starts.
A related topic encompasses the design of reliable policy rules.Age dependent and glide path rules are prominent examples for retirement plans. The previous DNN models are extraordinarily difficult to interpret. To improve the chance of implementation will require some type of translation into explainable policy rules. Similar issues arise in other ML domains and research is underway to find solutions.
Another area involves developing future projections in multi-period settings that depict historical patterns. One of the stylized facts is the contagion and high volatility that occurs during crash periods, as compared with normal economic conditions. Methods such as hidden Markov models, trend filtering, and recession detection algorithms can be applied to these issues, e.g. see reference [7].
4. Challenges
There are challenges to implementing machine learning to improve investment decisions. Certainly, machine learning can be helpful. The collection of more accurate data at the micro-level will assist in estimating risks and potentially increase profits for FinTech firms.At the same time, we are a long way from automated strategic decision systems, such as large investments. The path from classification (supervised learning) to strategic decision-making is a long winding way away, or so it seems at present.
Part of the difficult involves the lack of expertise on machine learning concepts by financial professional. To improve the situation, Professor Lionel Martellini and I have put together a massive online, open course (MOOC) entitled “Investment Management with Python and Machine” over the Coursera network [4].
Another barrier/challenge is the evolving nature of financial markets and the expansion of securities with complex structures. The discovery of micro factors for equities is complicated by the fact that a pattern, once discovered, can lose its power to outperform.An example is the famous “turn of the year” affect. This pattern is due to the asymmetric conditions by individuals (who pay taxes) and many institutions such as pension plans who do not pay taxes directly. The pattern has experienced lower returns since it was widely publicized. In some ways, the affect mimics quantum mechanics. It is a challenge to distinguish patterns that are durable from those from transient and to identify when and where patterns occurs.Likewise, the widespread use of common decision-making tools will have an unknown impact on markets as a whole.
A further challenge relates to the availability of micro-level data. As suggested, an equivalent gold rush is taking place to discover and mine micro-level data. However, due to its importance, many firms jealously guard the data, and thus researchers and policy makers are excluded from employing or even seeing the data. This limits future applications.Also, due to privacy and sensitivity concerns, data is often disguised and “adjusted” by means of complex algorithms. Imputation can only go so far. The area of health care is much affected by these conditions, and investment applications have similar barriers.
5. Outlook
The excitement regarding the future prospects for applying machine learning concepts in investment management is mostly warranted. One of the obvious applications involves collecting close-to-real time micro-level data in a quest to predict upcoming economic events, such as recessions or bank runs. In a similar way, agent based models (focused on individual decisions) could improve the spread of information and dynamics of markets.
Another area with a high probability of success is estimating risks at the personal level. Here again, micro-level data can be employed, such as collecting information on specific individuals, or to estimate the risks for loans. It seems likely that U.S. banks will greatly reduce the large number of branches in the future, with the emergence of alternative sources for loans via FinTech firms and with online lending slowly being accepted by traditional and shadow banks.
Another major domain for improvement is to assist individuals with their financial affairs in an integrated manner. Most people are faced with long term critical decisions about saving, spending, and investing to achieve a wide variety of goals. These decisions are often made without much professional guidance (except for wealthier clients), and without much technical training. Current personalized advisors are reasonable initial steps. Much more can be done in this area with modern data science and decision-making tools. Plus, younger people or more willing to trust fully automated computational systems. To my mind, this domain is the most relevant and significant for future investment management.
References
[1] Amenc, N. and F. Goltz (2016). Long-Term Rewarded Equity Factors: What Can Investors Learn from Academic Research? Journal of Index Investing 7 (2), 39–56.
[2] Amenc, N., F. Goltz, A. Lodh and L. Martellini. 2014. Towards Smart Equity Factor Indices: Harvesting Risk Premia Without Taking Unrewarded Risks. The Journal of Portfolio Management 40(4): 106–122.
[3] Arnott, R., C. Harvey, V. Kalesnik, J. Linnainmaa, “Alice’s Adventures in Factorland: Three Blunders that Plague Factor Investing,” Research Affiliates Report, 2019.
[4] Martellini, L. and J. Mulvey, “Machine Learning for Investment Management,” Coursera, 2019.
[5] Martellini, L., and V. Milhau, “Smart Beta and Beyond: Maximising the Benefits of Factor Investing,” EDHEC-Risk Institute Research Report, February 2018.
[6] Mulvey, J. and. M. Holen, “The Evolution of Asset Categories: Lessons from University Endowments”, Journal of Investment Consulting, Fall 2016, 17, 2, 48-58
[7] Mulvey, J. and H. Liu, “Identifying Economic Regimes: Reducing Downside Risks for University Endowments and Foundations,” Journal of Portfolio Management, 43, 1, 100-108, 2016.
[8] Mulvey, J., Y. Sun, M. Wang, and J. Ye, “Optimizing a Portfolio of Mean-Reverting Assets with Transaction Costs via a Feedforward Neural Network,” Princeton University working paper, 2018.