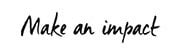
Industry Analysis
Multi-Asset Products and Solutions
Vincent Milhau
Within the old paradigm, the asset management industry had long focused on the design of products with attractive performance, in a context where the distinction between active management with high fees and the passive replication of a benchmark at more moderate fees was a structuring frontier. At least since the turn of the millennium, profound changes have been taking place, which have led to the value proposal for investment management being entirely rethought. The first of the changes is the increasing interest from investors for “smart beta” products, which give them access to investable risk premia at much lower costs than actively managed funds. The factor investing approach is an important aspect of this trend, because these premia can be interpreted as compensation for bearing exposure to undiversifiable risk factors. The second paradigm change is perhaps even more important and consists in the recognition that investors, be they individuals or big institutions, need dedicated (customised or mass-customised) solutions to achieve their goals, as opposed to off-the-shelf products.
Modern portfolio theory can serve as a useful guide towards the design of meaningful solutions, by teaching us what the right building blocks are, and how they should be combined over time to improve investor welfare. In practice, it will be needed, of course, to deviate from theoretical optimal portfolios to satisfy implementation constraints, but at least the key desirable properties of the building blocks and the allocation rule can be accounted for when defining implementable solutions. In particular, fund separation theorems state that optimal strategies combine at least two blocks: a performance-seeking portfolio with the highest possible Sharpe ratio, and a liability or goal-hedging portfolio that should track the present value of an investor’s liabilities or goal. This distinction has important implications when it comes to a better understanding of multi-asset solutions, since it implies that good risk-adjusted performance is only one possible objective – admittedly, one that is far from obvious to reach – for such multi-asset products, and the focus of a multi-asset solution should extend beyond this simple objective.
Scientific Diversification in Performance-Seeking Portfolios
The maximum Sharpe ratio (MSR) portfolio, defined as the portfolio that maximises the expected excess return over the risk-free rate per unit of risk taken, is a fundamental tenet of modern portfolio theory. It appears in (i) the two-fund separation theorem of Sharpe’s (1964) Capital Asset Pricing Model, (ii) dynamic portfolio strategies designed to strike the best balance between expected return and risk (Merton, 1973), (iii) optimal liability-driven investing strategies for pension funds or insurance companies (Martellini and Milhau, 2012), and (iv) goal-based strategies that maximise the probability of reaching a target wealth level (Browne, 1999).
While conceptually straightforward, the prescription to hold the MSR portfolio has proved extremely difficult to implement in practice, because of the need to estimate expected returns and covariances and the dramatic impact of estimation errors on out-of-sample performance. Estimation risk is so large that it can offset the expected benefits of scientific diversification, and the “naive” diversification rule that weights all constituents equally proves to be a not-so-easy-to-outperform benchmark (DeMiguel, Garlappi and Uppal, 2009). In view of this problem, it is tempting to use a portfolio construction technique that does not rely on expected returns, which are more the most difficult parameters to estimate.
The global minimum variance (GMV) portfolio is attractive from that perspective because it is the only point of the efficient frontier that depends solely on covariances, and it can be justified as an MSR portfolio under the agnostic assumption that all expected returns are equal. Moreover, in asset allocation, the number of constituents is typically small, so the sample size is sufficiently greater than the universe size to make sample risk in the covariance matrix limited (Kan and Zhou, 2007). Were constituents too numerous with respect to available samples, efficient estimation procedures, such as statistical shrinkage, are available to obtain a more robust parameter estimate. However, it should be noted that even with a perfectly estimated covariance matrix, a GMV portfolio has no reason to deliver an attractive Sharpe ratio because its objective is to minimise risk, regardless of the opportunity cost in terms of performance. Moreover, it tends to be concentrated in the least volatile asset classes, like Government bonds, so it does not accurately reflect an intuitive idea of what a “well-diversified portfolio” should look like.
Risk and Factor Allocation
To construct a well-diversified portfolio, one may go back to conventional wisdom, which advocates to “spread eggs across many baskets”. But what are eggs and baskets in asset allocation? The equal-weighting rule answers that eggs are dollars and baskets are constituents. But it is well known that a 50%-50% stock-bond portfolio, while being perfectly diversified in terms of dollar contributions, is poorly diversified in terms of risk contributions because its volatility is largely dominated by equity risk, which is by far the most volatile asset class. Risk parity has become a popular approach to correct for such imbalances since its goal is to ensure that all constituents (baskets) have the same contributions to risk (eggs) in the portfolio. In particular, this avoids excessive concentration in assets with low volatilities, even though such constituents still tend to have the largest weights.
This methodology, however, has its own limits. The first is inherent to the use of volatility as a risk measure: while volatility is symmetric and equally penalises upside potential and downside risk, investors are clearly more averse to downside risk than to uncertainty on the upside. As a result, standard forms of multi-class risk parity portfolios tend to overweight bonds, even in an environment where interest rates are expected to go up from historically low values to higher levels and bonds have a high downside risk. The adaptation of risk parity portfolios to interest rate conditions is the focus of a research paper by Martellini, Milhau and Tarelli (2015), supported by Lyxor in the context of the “Risk Allocation Solutions” research chair at EDHEC-Risk Institute. In this paper, we argue that a “duration-based volatility” measure for bonds, equal to current duration multiplied by interest rate volatility, more adequately captures increasing risk as interest rates decrease (see Exhibit 1). Extensions of the risk parity methodology to non-symmetric risk measures, such as semi-variance (the variance restricted to negative returns), value-at-risk or expected shortfall, are also discussed in this paper and in a related effort by Roncalli (2013). Users of these latter methods should be aware that they bear the risk of estimation errors in expected returns (required for downside risk estimates), but not as much as by attempting to estimate the MSR portfolio, because under suitable mathematical conditions given by Roncalli (2013), the extended risk parity portfolio is long-only, unlike the MSR portfolio, which can completely rule out assets with perceived unattractive risk-return profiles.
Exhibit 1: Yield-to-redemption and volatility estimates for a bond index (January 1973 – December 2012).
Source: Martellini, Milhau and Tarelli (2015).
The second limitation of the risk parity approach is that by focusing on the contributions of constituents, it completely disregards the underlying factors that explain the risk of constituents, which can lead to a misleading picture for correlated constituents with a strong common factor. For instance, an equally-weighted portfolio of two bonds with similar durations spreads dollars evenly, and is almost at risk parity, because the two volatilities are close to one another. Yet, the risk of this portfolio is related to a single factor bet, which is a bet on the level of interest rates. To better assess the extent of diversification in a multi-class portfolio, Carli, Deguest and Martellini (2014) propose to look through the constituents and to consider the risk contributions of underlying systematic factors. Implicit factors, which are extracted by analysing the returns of the securities to explain, are well suited for this purpose because they are uncorrelated, and they exhaust by definition all uncertainty over asset returns, in contrast to macroeconomic factors, which generally have low explanatory power. The effective number of uncorrelated bets that the authors use was introduced in Deguest, Martellini and Meucci (2014) to quantify the degree of diversification as a quantitative measure of the dispersion of risk contributions (eggs) across factors (baskets). In the previous example, it would be close to 1 because portfolio risk is almost entirely explained by the interest rate level factor.
The factor perspective in the construction of well-diversified portfolios is gaining popularity because it is theoretically supported by asset pricing models, and because it makes intuitive sense. As Ang (2014, p. 195) puts it, “factors are to assets what nutrients are to food”, so it is important for investors to be informed about what an appropriate diet is before deciding how to collect these nutrients. Moreover, factors go a long way towards explaining returns on long-only actively managed portfolios, as Ang, Brandt and Denison (2014) show in their study of the Norwegian sovereign wealth fund. Smart beta products, which have been largely developed in the equity class, are investment vehicles that allow factor premia to be collected at low costs and have made the distinction between active and passive management less clear (see the contribution on smart beta in the equity space in this special issue).
From Investment Products to Investment Solutions
A well-diversified "risky" performance-seeking portfolio is only one piece of the asset allocation process, albeit an important one. A multi-asset multi-factor solution should also involve a "safe" building block, as well as a suitably chosen allocation strategy to the risky and safe building blocks. The ultimate objective is to offer a high probability of reaching the investor’s aspirational goals while securing their essential goals, defined as minimum levels of wealth or consumption. Clearly, such a value proposal is very different from delivering a high alpha or outperforming a given benchmark, and this is precisely why investment solutions cannot be based on diversification principles alone.
The second important component for a meaningful solution is a safe liability- or goal-hedging portfolio (LHP/GHP), the role of which is to replicate the present value of a process that characterises an investor’s objectives which are summarised in terms of their liabilities in an institutional money management context, or their goals in an individual money management context. For an individual saving for retirement, this process is given by the discounted value of the future expected replacement income cash flows. To construct a LHP/GHP, a factor approach is also helpful, provided the risk factors that impact the liability value are identified. Interest rates are almost always a major source of risk due to the discounting mechanism, but inflation risk is also often present in situations where the target wealth level or income stream are inflation-linked. Thus, real bonds or nominal bonds are typically important ingredients of the LHP/GHP. Duration and convexity hedging, which are traditionally employed to hedge interest rate risk, are examples of factor-matching techniques used in this context.
Other building blocks may be necessary, as posited by Merton’s (1973) fund separation theorem: when investment opportunities (expected returns and volatilities) vary over time, “hedging portfolios” are required, which enjoy higher returns when market conditions get worse. For instance, bonds perform well when interest rates decrease, and stocks tend to have higher returns when the prospective equity premium is lower.
The final step in the design of investment solutions is the suitable allocation to the blocks. If the investor’s objective is to achieve the best trade-off between expected return and risk without any explicit target wealth or consumption level, Merton (1973) shows that the optimal allocation not only depends on the investor’s horizon and risk aversion, but also on the current values of risk premia. Target-date funds with a deterministic glide path are not a satisfactory implementation of these prescriptions, if only because they do not react to changes in market conditions (see the contribution on improved forms of target date funds in this special issue). Research has shown that enhanced long-term investing strategies based on these principles and satisfying realistic implementation constraints can be designed, which lead to higher welfare (Martellini and Milhau, 2010).
When the investor explicitly states wealth or consumption goals, meaningful investment solutions should maximise the probabilities of reaching them in favourable scenarios, and/or minimise the expected shortfall in those scenarios where market conditions do not make it possible for the goals to be reached. This multi-asset investment framework requires the use of risk-budgeting techniques, in which the allocation to the various building blocks is a function of the current distance to the goals. These methods have long been employed in portfolio insurance, but they should also be applied in a goal-based investing context. An extensive review of these strategies is done by Deguest et al. (2015) in a research paper supported by Merrill Lynch Wealth Management, and the contribution by Kévin Giron and Lionel Martellini on retirement goal indices in this issue describes an application to the retirement investing problem.
As a conclusion, investors do need multi-factor products designed to efficiently harvest risk premia across and within asset classes to generate attractive risk-adjusted performance. That said, they also need hedging building blocks and dynamic investment solutions products that efficiently allocate to the various blocks in such a way as to deliver high probabilities of reaching their meaningful goals.
This article is one of many articles in Investment Management Review (IMR) special edition Autumn 2017 which covers important recent insights into the asset management industry. To access the full edition please click on http://www.imrmagazine.com/edhec-special-edition.php.
References
Ang, A. 2014. Asset Management: A Systematic Approach to Factor Investing. Oxford University Press.
Ang, A., M. Brandt and D. Denison. 2014. Review of the Active Management of the Norwegian Government Pension Fund Global. Technical Report.
Browne, S. 1999. Reaching Goals by a Deadline: Digital Options and Continuous-Time Active Portfolio Management. Advances in Applied Probability 31(2): 551-577.
Carli, T., R. Deguest and L. Martellini. 2014. Improved Risk Reporting with Factor-Based Diversification Measures. EDHEC-Risk Institute Publication.
Deguest, R., L. Martellini and A. Meucci. 2014. Risk Parity and Beyond – From Asset Allocation to Risk Allocation Decisions. EDHEC-Risk Institute Working Paper.
Deguest, R., L. Martellini, V. Milhau, A. Suri and H. Wang. 2015. Introducing a Comprehensive Risk Allocation Framework for Goals-Based Wealth Management. EDHEC-Risk Institute Publication.
DeMiguel, V., L. Garlappi and R. Uppal. 2009. Optimal versus naive diversification: How Inefficient is the 1/N Portfolio Strategy? Review of Financial Studies 22(5): 1915-1953.
Kan, R. and G. Zhou. 2007. Optimal Portfolio Choice with Parameter Uncertainty. Journal of Financial and Quantitative Analysis 42(3): 621-655.
Martellini, L. and V. Milhau. 2012. Dynamic Allocation Decisions in the Presence of Funding Ratio Constraints. Journal of Pension Economics and Finance 11(4): 549-580.
Martellini, L. and V. Milhau. 2010. Towards the Design of Improved Forms of Target-Date Funds. Bankers, Markets and Investors 109: 6-24.
Martellini, L., V. Milhau and A. Tarelli. 2015. Towards Conditional Risk Parity – Improving Risk Budgeting Techniques in Changing Economic Environments. Journal of Alternative Investments 24(3): 5-29.
Merton, R. 1973. An Intertemporal Capital Asset Pricing Model. Econometrica 41(5): 867-887.
Roncalli, T. 2013. Introducing Expected Returns in Risk Parity Portfolios: A New Framework for Strategic and Tactical Asset Allocation. SSRN working paper.
Sharpe, W. F. 1964. Capital Asset Prices – A Theory of Market Equilibrium under Conditions of Risk. Journal of Finance 19(3): 425-442.