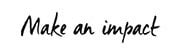
Industry Analysis
A Portfolio Perspective on the
Multitude of Firm Characteristics
Victor DeMiguel
Alberto Martin-Utrera
Francisco J. Nogales
Raman Uppal
Hundreds of variables have been proposed to predict the cross-section of stock returns; see, for instance, Harvey, Liu, and Zhu (2015), McLean and Pontiff (2016), and Hou, Xue, and Zhang (2017). This large number of cross-sectional predictors leads Cochrane (2011) to ask, "Which characteristics really provide independent information about average returns? Which are subsumed by others?" Cochrane challenges researchers to characterize the dimension of the cross-section of stock returns by identifying a small set of characteristics that subsume the rest.
To address this challenge, several recent papers use cross-sectional regressions to shrink the cross section of expected stock returns; see, for instance, Green, Hand, and Zhang (2017), Freyberger, Neuhierl, and Weber (2016), and Messmer and Audrino (2017). These papers study the dimension of the cross section in the absence of transaction costs, but transaction costs matter because the hundreds of predicting variables discovered in the literature pose a challenge to the efficient market hypothesis only if investors can exploit them to build a portfolio that delivers superior risk-adjusted returns net of transaction costs. To address this gap in the literature, we study how transaction costs affect the dimension of the cross section of stock returns.
To achieve our objective, we study how many firm-specific characteristics matter jointly from a portfolio perspective; that is, from the perspective of an investor who cares not only about average returns, but also about portfolio risk and transaction costs. A portfolio perspective is required in order to assess how many characteristics matter jointly because it is optimal to trade combinations of characteristics to reduce both portfolio risk and transaction costs.
Our main finding is that transaction costs significantly increase the dimension of the cross section of stock returns. We consider a dataset with 51 characteristics and we find that while in the absence of transaction costs only six are significant, in the presence of transaction costs this number increases to 15. The intuition behind this result is that combining characteristics is advantageous in the presence of transaction costs because the trades in the underlying stocks required to rebalance different characteristics often cancel each other out, and thus, combining a larger number of characteristics allows one to substantially reduce transaction costs. Essentially, combining characteristics allows one to diversify trading, just as combining them allows one to diversify risk.
To undertake our analysis, we extend the parametric portfolio framework in Brandt, Santa-Clara, and Valkanov (2009). Parametric portfolios are obtained by adding to a benchmark portfolio a linear combination of the long-short portfolios associated with each of the firm-specific characteristics considered. Then, we address three research questions. First, how many characteristics are jointly significant from a portfolio perspective? Second, how does the answer to this question change with transaction costs? Third, can an investor improve out-of-sample performance net of transaction costs by exploiting a large set of characteristics instead of the small number considered in prominent asset- pricing models?
Our answers to these three questions are as follows. First, in the absence of trans- action costs, only a small number of characteristics - about six - are significant. Five characteristics -unexpected quarterly earnings, return volatility, asset growth, 1-month momentum, and gross profitability - are significant because they increase the mean return and also help to reduce the risk of the portfolio of characteristics. A sixth characteristic – beta - is significant only because of its ability to reduce the risk of the other characteristics, in particular, the return-volatility characteristic. The returns of the beta and return-volatility characteristics are highly correlated over time, but while return volatility has a large (negative) mean return, beta has a negligible mean return. Thus, going long the beta characteristic allows the investor to hedge the risk of her short position in the return-volatility characteristic, without compromising its mean return. We find that traditional characteristics such as 12-month momentum and book to market are not significant because, although they have high mean returns, they do not offer a sufficiently attractive tradeoff between portfolio mean return and risk.
Second, in contrast to what one would find if evaluating characteristics in isolation, we find that the presence of transaction costs increases the number of jointly significant characteristics from six to 15. This is because the benefits of trading diversification are large when combining characteristics optimally. Indeed, we find empirically that the marginal transaction cost of trading the stocks underlying a characteristic is reduced by around 65% on average when they are combined in an optimal parametric portfolio. Consequently, certain characteristics that would require a large amount of trading in the underlying stocks if exploited in isolation, such as the short-term-reversal characteristic (i.e., 1-month momentum), continue to be significant in the presence of transaction costs because of the trading diversification possible from combining characteristics.
Finally, we show that an investor can exploit a large set of characteristics in the presence of transaction costs to achieve an out-of-sample Sharpe ratio that is larger than that obtained by exploiting small sets of characteristics that are typically considered in prominent asset-pricing models. For instance, we find the investor achieves an out-of- sample Sharpe ratio of returns net of transaction costs around 100% larger than that from exploiting the three traditional characteristics considered in Brandt, Santa-Clara, and Valkanov (2009) and 25% higher than that from exploiting a set of four characteristics that include investment and profitability characteristics, which are highlighted in Hou, Xue, and Zhang (2014) and Fama and French (2015). These out-of-sample results confirm that in the presence of transaction costs the cross section of stock returns is not fully explained by a small set of characteristics.
Our work demonstrates that accounting for transaction costs increases the number of characteristics that are significant for an investor, and thus, transaction costs provide an economic rationale for non-sparse characteristic-based asset-pricing models.
Full publication is available here for download
References
Brandt, Michael W., Pedro Santa-Clara, and Rossen Valkanov, 2009, Parametric portfolio policies: Exploiting characteristics in the cross-section of equity returns, Review of Financial Studies 22, 3411-3447.
Cochrane, John H., 2011, Presidential address: Discount rates, The Journal of Finance 66, 1047-1108.
Fama, Eugene F., and Kenneth R. French, 2015, A _ve-factor asset pricing model, Journal of Financial Economics 116, 1-22.
Freyberger, Joachim, Andreas Neuhierl, and Michael Weber, 2016, Dissecting characacteristics nonparametrically, Chicago Booth working paper.
Green, Jeremiah, John R.M. Hand, and X. Frank Zhang, 2017, The characteristics that provide independent information about average us monthly stock returns, Forthcoming in The Review of Financial Studies.
Harvey, Campbell R., Yan Liu, and Heqing Zhu, 2015, ...and the cross-section of expected returns, The Review of Financial Studies 29, 5-68.
Hou, Kewei, Chen Xue, and Lu Zhang, 2014, Digesting anomalies: An investment approach, Review of Financial Studies 28, 650-705.
Hou, Kewei, Chen Xue, and Lu Zhang, 2017, Replicating anomalies, Technical report, National Bureau of Economic Research.
McLean, R. David, and Jeffrey Ponti_, 2016, Does academic research destroy stock return predictability?, The Journal of Finance 71, 5{32.
Messmer, Marcial, and Francesco Audrino, 2017, The (adaptive) lasso in the zoo- firm characteristic selection in the cross-section of expected returns, University of St.Gallen working paper.
About the authors
DeMiguel, London Business School, e-mail: [email protected];
Martin-Utrera, Lancaster Uni- versity Management School, e-mail: [email protected];
Nogales, Universidad Carlos III de Madrid, e-mail: [email protected];
Uppal, Edhec Business School and CEPR, e-mail: [email protected].