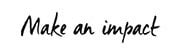
Nicole Beevers, Quantitative Strategist, Rand Merchant Bank, a division of FirstRand Bank Limited; Hannes Du Plessis, Quantitative Strategist, Rand Merchant Bank; Lionel Martellini, Professor of Finance, EDHEC Business School, Director, EDHEC-Risk Institute; Vincent Milhau, Research Director, EDHEC-Risk Institute
Merton’s (1973) fund separation theorem establishes that there are at least two reasons why an investor would want long or short exposure to a given risky asset. The first is the “speculative motive”, which is to maximize the short-term Sharpe ratio of the portfolio, and it drives the introduction of the maximum Sharpe ratio (MSR) portfolio. The second is the “hedging motive”, which is to hedge against unfavorable changes in investment opportunities, e.g. interest rates or risk premia. In the presence of liabilities, a third motive exists, which is to hedge against unexpected changes in the value of liabilities (Martellini and Milhau, 2012), so a liability-hedging portfolio (LHP) enters the solution as an additional building block. The introduction of minimum performance constraints, such as a minimum wealth or funding requirement in asset and liability management impacts the allocation decision regarding these funds. For instance, the optimal strategy in the presence of a minimum wealth constraint involves a dynamic allocation between the performance-seeking portfolio (PSP) and a pure discount bond that pays off the desired minimum (which represents the hedging demand against unexpected changes in interest rates), and the outcome of that strategy is a non-linear payoff equal to the payoff of a bond-plus-call strategy. The optimal strategy is thus a form of option-based portfolio insurance (El Karoui, Jeanblanc and Lacoste, 2005). Deguest, Martellini and Milhau (2014) extend this result to asset-liability management by showing that the optimal liability-driven investing strategy in the presence of a minimum funding ratio constraint involves dynamic allocation to the PSP and the LHP.
The above fund separation theorems describe the building blocks and the allocation rule to be used to maximize expected utility, but there are situations in which some of the building blocks and/or the allocation strategy are given and cannot be optimally chosen. This is in particular the case in delegated portfolio management contexts, where each decentralized asset manager is tasked with managing a sub-component of the whole investor portfolio, with a payoff function that is exogenously fixed and not necessarily optimal.[1]
If the PSP is not meant to be used naked but as part of an investment strategy that involves one or more other building block(s), then how should it be constructed? It is unclear whether the standard Sharpe ratio maximization prescription is optimal for each sub-component of the portfolio even if it is at the overall portfolio level. Besides, even if the manager is in charge of the whole PSP, a pure focus on the expected return and the volatility of their portfolio is only rational in the absence of liabilities. For liability-driven investors, relative risk is a more meaningful objective. Moreover, preferences are not always accurately represented by the simple mean-variance utility function, and other welfare criteria which capture higher-order moments, like expected utility, or explicitly penalize downside risk, like expected shortfall, may be regarded as more appropriate.
To address these questions, this paper provides a characterization for the optimal PSP for a given welfare function when this PSP is used with another building block in a multi-asset portfolio that may involve rebalancing. As in Merton (1973) and Cox and Huang (1989), we are interested in analytical expressions of optimal portfolios, since these expressions facilitate numerical calculations, hence practical implementation, and they help understand the impact of exogenous parameters, such as the PSP’s weight in a fixed-mix strategy or the minimum wealth level in an insurance strategy. Fully explicit solutions are not always available in extremely general settings, but in most of the cases presented in this paper, the welfare function can be calculated explicitly, which makes numerical optimization fast and accurate.
We demonstrate that the optimal PSP can be represented as a combination of several funds, which involves an MSR portfolio plus one or more other fund(s). This result implies that the MSR portfolio alone is in general suboptimal. Among the other funds are the global minimum variance (GMV) portfolio and the “most liability-friendly” (MLF) portfolio, which maximizes the correlation with liabilities. The allocation to the various funds depends on the welfare function and the strategy in which the PSP is used.
This personalized approach to PSP construction is somewhat similar to the precision medicine model, widely regarded as a fundamental breakthrough that will mark the start of a whole new era for medical practice by proposing the customization (or mass-customization) of healthcare, with treatments, practices or products being tailored to a subgroup of patients, instead of a one-drug-fits-all model. For this reason, we use the term precision investing to define a personalized investment strategy that is tailored to optimize investor suitability versus standalone risk-adjusted performance.
Let us start with a two-step investment process in which a centralized decision maker chooses an insurance strategy whose payoff is a convex function of the PSP payoff, and a decentralized asset manager is assigned the task of constructing a PSP. The optimization problem is to search for the optimal PSP for a given payoff function.
Beginning with some initial wealth or asset level at time 0, the insurance strategy aims to secure minimum wealth level M by time T by investing in a PSP and a pure discount bond that pays $1 at time T. To obtain a closed-form expression for the optimal PSP, we need to make a few stylizing assumptions:
OBPI involves purchasing a pure discount bond that pays M at time T, plus n European call options written on a PSP with value S. By imposing that the strike price be equal to M/n, the payoff of this strategy is . The number of options is then determined by the budget constraint, which states that initial wealth is split between the bond and the options. In those scenarios where the options end in the money, the relative gross return of the insured portfolio with respect to the PSP is
, a quantity that we call “access to upside” because it is the fraction of the PSP return that is captured with the insurance strategy.
is always less than 1, reflecting the fact that insurance against downside risk has a strictly positive opportunity cost. A noteworthy property of
is that it is decreasing in the PSP volatility, as can be seen by rewriting terminal asset value as a bond-plus-call payoff,
. A lower PSP volatility implies a lower call price for a given spot price, so
must increase for the call price to stay constant.
With CPPI, insurance is achieved by taking the dollar allocation to the PSP at each point in time to be a constant multiple m of the risk budget, where the risk budget is defined as the distance between the current portfolio value and the floor. The floor is the discounted value of the minimum target wealth level.
The standard optimization criterion in the academic literature is the expected utility from terminal wealth, that is for some utility function U. The power utility function,
, is a standard choice. When the risk aversion parameter Y is set to zero, the welfare metric is risk-neutral and reduces to expected wealth. Unlike the quadratic utility function that is used in mean-variance portfolio analysis, expected power utility captures the impact of higher-order moments (most notably skewness and kurtosis) on welfare.
The value of expected utility has no economic significance, so the agent in charge of constructing the PSP may prefer to use goal-based investing criteria, e.g. maximizing the probability of reaching a target wealth level or minimizing the expected shortfall with respect to that level. Mathematically, the success probability and the expected shortfall given a target wealth N are written as and
. It can be noted that minimizing the expected shortfall is equivalent to maximizing the expectation of a concave function of wealth, so this objective is qualitatively similar to expected utility maximization.
Under the assumptions of geometric Brownian prices and a fixed-mix PSP, the PSP payoff is log-normally distributed, so all the above welfare metrics can be written as functions of the expectation and variance of the logarithmic PSP return, respectively denoted by
and
. This property holds more generally with any welfare criterion that can be written as
for some function
. Explicit expressions for the function f are given in the Appendix.
The optimal vector of percentage weights in the risky assets is given by the following proposition.
Let
and assume that at the optimum. Then, the optimal PSP is
MSR and GMV respectively denote the maximum Sharpe ratio portfolio and the global minimum variance portfolio, and are the Sharpe ratio and the volatility of the MSR portfolio. All vectors have length equal to the number of constituents.
The two funds that make up the optimal PSP are the standard building blocks of mean-variance analysis, namely the maximum Sharpe ratio (MSR) and global minimum variance (GMV) portfolios, and the weights of these funds depend on the function f, hence on the welfare metrics and the shape of the insured payoff. The representation given by Proposition 1 is not completely explicit because the optimal weight vector is present in the right-hand side through the derivatives of f. But it is an equation that can be numerically solved for w*, and this numerical routine is fast and accurate thanks to the above analytical expressions.
Table 1: Utility-maximizing performance-seeking portfolios for insured payoffs
To give concrete examples of optimal PSPs, we consider the problem of optimizing the stock portfolio that serves as the underlying asset of an insurance strategy (OBPI or CPPI). Because the manager in charge of the PSP ends up holding two portfolios, namely MSR and GMV, it suffices to assume that she has access to two risky assets, which respectively correspond to the two portfolios. Deguest, Martellini and Milhau (2021) show that the Sharpe ratios (λ),volatilities and correlation
of the MSR and GMV portfolios satisfy the equalities
We let . These values imply
= 60% and
=0.24. Assuming a 1% short-term rate, we obtain expected returns of 9% and 3.88% per year for the MSR and GMV portfolios.
The risk aversion parameter in utility (y) is set to 10, the investment horizon is one year, and the target wealth in expected shortfall is taken to be with
. Thus, the target is 110% of the amount of wealth that would be attained by investing in cash. We set the floor as a minimal percentage of that amount, which ranges from 10% to 90%. A 0% floor would correspond to no insurance, and a 100% floor implies that initial wealth is fully invested in cash, so that the choice of the PSP is irrelevant. Optimal PSPs are calculated by numerically maximizing expected utility or by minimizing the expected shortfall. Analytical expressions for the derivatives of the welfare function are provided to the optimizer to accelerate convergence.
Our benchmark PSP is the MSR portfolio, which would be the default choice for an investor endowed with mean-variance preferences, seeking a portfolio fully invested in risky assets and ignoring the payoff function. The utility gain of the optimal PSP with respect to the MSR portfolio is measured as the “monetary utility gain”, which is the quantity denoted with MUG such that investing in the insurance strategy with the MSR portfolio delivers the same utility as investing
in the strategy with the optimal PSP.
The numerical results are shown in Tables 1 and 2. Although leverage constraints have not been introduced, the chosen parameter values imply long-only allocations in many cases. With the utility criterion, both weights are positive for floors ranging from 10% to 80%, and with expected shortfall, they are positive until a 60% floor level. With both criteria, the optimal share in the MSR portfolio is increasing in the floor: with expected utility and a CPPI multiplier of 3, the MSR allocation is 8.42% for a 10% floor and grows to 16.29% for a 50% floor and to 60.09% for a 90% floor, with the remainder invested in the GMV portfolio. With OBPI, the same qualitative pattern is observed, but the MSR weight ranges within narrower bounds, from 20.00% to 34.78% with utility-based preferences and from 42.93% to 56.13% with shortfall-based preferences. Remarkably, the optimal PSP shows little sensitivity to the floor level while the floor ranges from 10% to 50%: it has 20% in the MSR constituent with expected utility and 42.93% with expected shortfall. This happens because for low floors, the probability for the non-insured PSP to fall short of the minimum is close to zero, so that the coefficient of access to upside, , is almost 1. In these specific circumstances, the optimal PSP coincides with the one that maximizes expected utility or minimizes expected shortfall, regardless of the non-linear payoff.
Not only is the optimal share of MSR asset increasing in the floor, but it is also decreasing in the multiplier of CPPI strategies, from 167.97% for a multiplier of 1 down to 31.43% for a multiplier of 5 with the shortfall criterion. Therefore, more conservative insurance strategies require a greater share of MSR portfolio to compensate for the larger amount of risk-free asset that is introduced at the asset allocation stage.
The welfare gains from optimizing the PSP mechanically increase with the allocation to the PSP, so they are lower at higher floors and with higher CPPI multipliers. For a 70% floor and a multiplier of 3, the monetary utility gain is 3.49% of initial wealth, but it grows to 10.03% for a 50% floor. For a 70% floor and a multiplier of 3, the expected shortfall decreases from 10.17% to 9.00%, and if the multiplier rises to 5, the decrease is from 13.94% to 11.15%.
In theory (Martellini and Milhau, 2012), utility-maximizing liability-driven investing (LDI) strategies involve two building blocks, namely the maximum Sharpe ratio portfolio and the portfolio that has the largest squared correlation with liabilities, and the allocation to these funds depends on risk aversion and also on risk budgets (distance to floor) if a minimum funding constraint is imposed. In practice, LDI falls under the class of two-step investment strategies, where a centralized manager decides how much to allocate to a PSP versus a liability-hedging portfolio (LHP), and decentralized managers are in charge of constructing the building blocks, not necessarily by following the Sharpe ratio maximization and the correlation maximization prescriptions. The optimization problem that we address now is the optimal choice of the PSP for a given (and not necessarily optimal) choice of LDI strategy and LHP.
Table 2: Expected shortfall-minimizing performance-seeking portfolios for insured payoffs
The simplest LDI strategies are of the buy-and-hold or fixed-mix types. They are characterized by a single parameter , which is the initial percentage weight allocated to the PSP. For mathematical tractability purposes, we assume that fixed-mix portfolios are continuously rebalanced, but we do not assume that the LHP perfectly matches liability returns, and we denote its value with B to make it distinct from L, the present value of liabilities.
Another, more sophisticated, class of LDI strategies is designed to keep the funding ratio above a certain minimum at all times, to comply with the requirements of a third party (e.g. a pension plan’s sponsor or the regulator) or simply as self-imposed discipline (see Martellini and Milhau (2012) for a derivation of optimal investment policies in the presence of such constraints).
The welfare metrics applied to insurance strategies are still relevant in asset-liability management, but they should now apply to the funding ratio R = A / L , as opposed to asset value A. Indeed, the quantity of interest is not the absolute value of assets but the level of assets relative to liabilities. Thus, the expected shortfall is now calculated with respect to a target funding ratio expressed as , that is a multiple
(greater than 1) of the initial funding ratio.
To derive as many analytical expressions as possible, the assumption of geometric Brownian motion dynamics is extended to the present value of liabilities. Under this condition, the welfare metrics can be written as , where
are the covariances of the PSP with liabilities and the LHP, respectively. The solution to the optimization problem is given in the following four-fund separation theorem.
Let
and assume that we have at the optimum. Then, the optimal PSP is
MLF and MBF respectively denote the “most liability-friendly” and the “most LHP-friendly” portfolios, which maximize the squared correlations with liability returns and LHP returns, respectively. is the beta of liabilities with respect to the MLF portfolio, and
is the beta of the LHP with respect to the MBF portfolio.
When the LHP B perfectly replicates liability returns L, the MLF and MBF portfolios are identical, so Proposition 2 reduces to a three-fund separation result. It should be noted that the MLF portfolio differs from the LHP in that it is invested in the PSP constituents only. Thus, if the PSP universe is formed within an equity universe but liabilities are bond-like, the LHP is typically a duration-matching fixed-income portfolio while the PSP is the most “bond-like” equity portfolio (see Coqueret, Martellini and Milhau, 2014).
Table 3: Optimal performance-seeking portfolios in liability-driven investing strategies
With the expected utility criterion and a fixed-mix strategy, we have a fully explicit expression:
In the numerical illustration, we assume that liabilities are similar to a long-term bond with an expected return of 2% per year and volatility of 4%. We assume here for simplicity that the LHP perfectly replicates the liabilities, so the manager in charge of the PSP holds the MSR, MLF and GMV portfolios. These portfolios are assumed to be fully invested in equities, with volatilities respectively taken to be 21%, 19% and 17%, and expected returns respectively set at 9.40%, 4.80% and 6.50%. The correlations (in percentage) are set as follows:
Table 3 displays examples of optimal PSPs for buy-and-hold and fixed-mix LDI strategies, with expected utility or expected shortfall as the welfare criterion. For any combination of an investment policy and a welfare function, the optimal share of MSR decreases as the PSP weight increases, while the MLF portfolio is assigned an increasing weight. The GMV weight tends to increase too, although non-monotonically. This result confirms that a combination of the MLF and the GMV portfolios acts as a (non-perfect) substitute for the LHP.
The weight assigned to the MLF portfolio is low overall, reaching 1.33% at most, but it strongly depends on the assumed correlation with liabilities (see Equation (1)), which is assumed to be a low 7% here. In additional tests, the results of which are not shown here, we have considered a stock-bond universe for the PSP, in which the MLF has 80% correlation with liabilities, and have found that the optimal share of MLF can exceed 30% when the PSP weight is greater than 50%.
This paper introduces a continuous-time framework for portfolio optimization that differs from Merton’s (1973) seminal model in two ways. First, optimization does not apply to the entire portfolio of an investor, but only to the “performance-seeking portfolio” (PSP) managed in isolation from the remainder of the portfolio, which is invested in other building blocks that are taken as given, e.g. cash in portfolio insurance or the liability-hedging portfolio in a liability-driven investing strategy. Also taken as an input is the investment policy combining the PSP with the other building blocks, which ranges from simple buy-and-hold policies to more sophisticated portfolio insurance strategies. Second, the welfare metric to be maximized is not necessarily the expected utility of the PSP payoff, but can be a general function of the value of assets, which depends on the payoffs of the other building blocks and the multi-portfolio investment policy. If the strategy involves rebalancing, the corresponding terminal asset value is a non-linear function of the PSP payoff, e.g. a concave function for fixed-mix policies or a convex one for portfolio insurance strategies. An important area for further research will be to relax the assumption of geometric Brownian motion for the prices of PSP constituents, but this framework already provides interesting insights: optimal PSPs can be written as combinations of the traditional maximum Sharpe ratio and global minimum variance (GMV) portfolios, plus a “most liability-friendly portfolio” (MLF), which maximizes the correlation with liabilities if welfare is derived from the relative value of assets with respect to liabilities. The optimal share of the proxy for the risk-free asset, which is either the GMV or MLF portfolio, decreases when the investment policy at the asset level gets more conservative.
Additional work is needed to design an operational framework for the practical implementation of optimal PSPs, including the choice of the PSP constituents and a suitable methodology to estimate their risk and return parameters. The analysis conducted in this paper suggests that investors would benefit from the availability of such “precision investing portfolios” tailored to their specific circumstances, as opposed to being left with portfolios that focus on standalone performance. Just like modern healthcare seeks “precision medicine” tailored to a patient’s personal situation, as opposed to using the same treatment for everyone, “precision investing” departs from the Sharpe ratio maximization paradigm to seek optimal PSPs that explicitly take into account an investor’s preferences and constraints. As such, it helps shift the emphasis away from investment products towards genuine investment solutions.
The research from which this article was drawn was produced as part of the EDHEC-Risk Institute/FirstRand research chair on Designing and Implementing Welfare-Improving Investment Solutions for Institutions and Individuals. [2]
Appendix
The expected utility and expected shortfall of an OBPI strategy are given by the following formulas:
In these formulas, denotes the cumulative distribution function of the standard normal distribution[3],
Footnotes
[1] That the portfolio eventually held by investors is not optimal can be explained by the presence of frictions preventing them from fully revealing their preferences to decentralized asset managers, or by the presence of frictions preventing asset managers from coordinating their actions so as to implement a utility-maximizing payoff.
[2] We are grateful to Albert De Wet for very useful feedback.
[3] This function is present in the Black-Scholes formula for the pricing of European options.
References
Coqueret, G., L. Martellini and V. Milhau. 2014. Equity Benchmarks with Improved Liability-Hedging Properties. Journal of Portfolio Management 43(2), 37–49
Cox, J. and C. Huang. 1989. Optimal Consumption and Portfolio Policies when Asset Prices Follow a Diffusion Process. Journal of Economic Theory 49(1), 33–83
Deguest, R., L. Martellini and V. Milhau. 2014. Hedging versus Insurance: Long-Horizon Investing with Short-Term Constraints. Bankers, Markets and Investors (Special Issue March-April 2014), 33–47
Deguest, R., L. Martellini and V. Milhau. 2021. A Characterization of the Funds in Fund Separation Theorems. Working paper
El Karoui, N., M. Jeanblanc and V. Lacoste. 2005. Optimal Portfolio Management with American Capital Guarantee. Journal of Economic Dynamics and Control 29(3), 449–468
Martellini, L. and V. Milhau. 2012. Dynamic Allocation Decisions in the Presence of Funding Ratio Constraints. Journal of Pension Economics and Finance 11(4), 549–580
Merton, R. C. 1973. An Intertemporal Capital Asset Pricing Model. Econometrica 41(5), 867–887