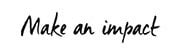
By Riccardo Rebonato, Scientific Director, EDHEC-Risk Climate Impact Institute, Professor of Finance, EDHEC Business School
This edito by Riccardo Rebonato, Scientific Director of EDHEC-Risk Climate, has been originally published in the October newsletter of the Institute. To subscribe to this complimentary newsletter, please contact: [email protected].
Climate change presents unprecedented challenges to investors, policy makers and regulators. Adaptation, avoidance and remedial action all require knowledge of what may lay ahead of us, but unfortunately, with climate change we cannot say that “we have been here before”. This is why climate scenario analysis (and its cousin, stress testing) must play a particularly important role in guiding our responses to climate change. As we explore this unchartered territory, we are faced with greater challenges than traditional financial stress testing presents because we have much less data to draw on and shaky models linking temperature increases to economic damage. Yet, despite these difficulties, we are forced to also do something we usually dispense with when handling macrofinancial scenarios: we must assign at least order-of-magnitude probabilities to the various climate occurrences.
The last statement may seem contentious, as financial scenario analysis is often conducted without explicit probabilities. When we look at climate change, however, the situation is radically different. Typically, when it comes to macrofinancial scenarios, we can draw on more than 100 years of crises, surprises and wild market gyrations. Even if no probability is explicitly assigned to a financial scenario, based on what has happened in the past we can make an estimate, in the back of our minds, as to its approximate severity. And if we really want, there are formal econometric techniques to gauge the blackness of financial swans. However, matters are totally different with climate scenarios, simply because we have precious little information about what damages climate change may inflict on the world’s economic system. To make the point more forcefully, the very plausible scenario of a 3°C warming by 2100 brings into play temperatures never yet experienced by the human species, let alone civilization.
And climate scenarios also present additional problems. With the macrofinancial system, we deal (or we assume that we deal) with a fundamentally stationary problem – perhaps with different regimes, but with a well-defined “transition matrix” connecting them. However, when we look at the impact of the climate on human societies, we see an intrinsically dynamic problem, and an adaptive one at that, because our responses will also act as both a cause and an effect on climate outcomes. This makes the problem vastly more difficult.
Yet, if we want to devote our costly and all too-limited-abatement and mitigation resources effectively, we need to know, at least approximately, the likelihood of different climate scenarios. Is 4°C by 2100 the stuff science fiction movies are made of, or does it constitute a “clear and present danger”? Without some probability estimates, we simply cannot say. And, as Sunstein (2005) eloquently argues in his critique of the unbridled precautionary principle, we cannot, and should not, attempt to take remedial action against anything that might happen. We must use our silver bullets wisely, and aim them at the most likely dangers.
Unfortunately, the current climate scenario framework (the SSP/RCP[1] approach) totally eschews any probabilistic statements whatsoever. Its architecture consists of “matching” a socioeconomic narrative with an emission schedule[2] and working out the “social cost of carbon” associated with that combination.[3] Given this matching, the SSP/RCP framework then relies on a chosen set of Integrated Assessment Models to find the one and only combination of macrofinancial variables that, according to the models, best describes the chosen combination of socioeconomic developments and emissions.[4] To mention just one disturbing omission among many of this approach, since only the most likely outcome is considered, and since most economists believe that the positive economic growth we have experienced in the last two and half centuries will continue to the end of the century and beyond, the possibility of zero (let alone negative) economic growth out to 2100 is not even contemplated. Even more worryingly, the possibility of a major economic recession is not on the cards either.[5]
Needless to say, since only the most likely combination of macrofinancial, technological and demographic variables is considered, with the SSP/RCP approach it does not even make sense to speak to distributions of outcomes: as a result, there are no distribution tails (fat or otherwise), and there is no uncertainty. With the SSP/RCP approach, we may not know which combination of emissions and socioeconomic developments will prevail but, given this information, we know absolutely everything about the economics, the technology development and the demographics of the world system. Focussing on the most likely outcome has a clear merit. However, humans do not make decisions under uncertainty only by looking at the most likely outcome.[6] And when this single quantity becomes the only piece of information provided, it can give rise to complacency and underestimation of the risks ahead of us. These worries become even greater when we observe that all the scenarios built by the Network of the Greening of the Financial System (NGFS) have been constructed taking as a starting point the socioeconomic narrative dubbed “Middle of the Road”.
The SSP/RCP framework has been built thanks to the efforts of dozens of very reputable academics, from equally reputable institutions. Since nothing of comparable quality existed before their efforts, these academics have rendered an invaluable service to the scientific community, and to society at large. Saying that something is ‘seminal’ or ‘pathbreaking’ doesn’t mean, however, that it cannot be improved upon. And this is exactly what the research efforts at EDHEC Risk Climate Impact Institute attempt to do: at least in one major research strand, we try to complement and build on the standard approach, and to remedy what we perceive to be the greatest weakness of the SSP/RCP approach – the absence of any probabilistic information.
Creating a probability distribution (as opposed to a point estimate) means being fully cognisant of, and able to capture, the very different sources of uncertainty that characterise the climate/economy system. Somewhat schematically, these can be summarised as follows:
Given the different nature of these four sources of uncertainty, different ways to handle model risk and intrinsic stochasticity are called for.[7] The first important point is that the whole approach displays a naturally modular structure, where the different parts (e.g., damage function, climate physics) can and should be modelled independently, with stand-alone ‘removable’ modules.
Even assuming that a modular scenario architecture is embraced, the type of output still requires careful analysis: if an emission schedule to 2100 is assigned, then it is possible to take the model uncertainty and the intrinsic stochasticity of the system into account using traditional statistical and econometric techniques (where ‘traditional’ should not be confused for a moment with ‘easy’ or ‘straightforward’) and to produce the sought-after distribution of outcomes. The problem remains dauntingly difficult, but then there exists, at least, an accepted map of the terrain.
What is much more difficult is to obtain unconditional probability distributions (of temperature, damages, economic averages, etc) – distributions, that is, that come from assigning appropriate weights to the different policy options. Yet, this is the piece of information that would be most useful to investors and policymakers. Even here, however, something informative can be said:
In short, as long as we are aware of the limitations of our knowledge, limited and imprecise information is better than no information at all – or, in slightly more formal terms, it is difficult to believe that a totally diffuse prior is the best way to characterise our state of knowledge about the climate/economy system.
When it comes to climate scenario analysis, an important part of the research efforts of the EDHEC Risk Climate Impact Institute is therefore currently focussed in the direction of creating full probability distributions for the possible climate outcomes: Kainth and Melin (2023) do so while trying to remain as close as possible to the SSP/RCP approach; Rebonato and Kainth (2023a) take a different approach, and depart more markedly from the standard framework; Rebonato and Kainth (2023b) review the SSP/RCP and the ‘modular’ approaches in some detail. These research efforts are part of a wider research project that EDHEC-Risk Climate is undertaking in the context of a new Research Chair with Scientific Beta, aptly entitled “Upgrading Climate Scenarios for Investment Management”. In all these cases, the underlying motivation is the same; a useful scenario analysis must satisfy two essential requirements at the same time: it must account for the full dispersion of possible outcomes (point estimates have some value, but can be misleading); and it must convey an approximate estimate of the relative probability of different climate outcomes. None of the approaches discussed above fully ‘solve’ the problem, but they are hopefully useful and sorely needed, steps in the right direction.
Footnotes
[1] The acronyms SSP and RCP stand for Socioeconomic Pathways and Representative Carbon Pathways, respectively.
[2] To be more precise, what is specified in the RCP part of the scenario construction is the forcing (difference in energy in and energy out) by the end of the century (expressed in Watt/m2). The forcing is affected, among other fixed factors, by the CO2 concentration, which is in turn a function of the emission trajectory.
[3] For a description and critical analysis of the SSP/RCP scenario approach, see Rebonato and Kainth (2023).
[4] For a discussion of how the SSP/RCP scenarios are constructed, see Rebonato and Kainth (2023).
[5] For a discussion of the plausible, but over-rigid, assumptions embedded in the SSP/RCP framework, see Rebonato and Kainth (2003).
[6] I am not just referring to a decisional criterion such as expected utility maximisation. Also other, more ‘frugal’ criteria, such as minimax or smooth ambiguity aversion, depend on the range of possible outcomes.
[7] Model risk arises when different models, of different reliability, attempt to describe the same phenomenon. If the phenomenon itself is then stochastic, even if we knew the true and correct model and all its parameters, we would still have residual variability.
[8] Given the current level of CO2 emissions (around 37 gigatonnes/year), and the world GDP (USD96.5 trillion), it is easy to work out that social costs of carbon of USD100/tonne, USD200/tonne and USD400/tonne would correspond to a global ‘carbon tax’ equivalent to 3.8%, 7.6% and over 15% of GDP. The latter percentage is more than what the world currently devotes to education, defence and medical care combined.
[9] For a discussion of the possible sources of information about the acceptability of various levels of the ‘implied social cost of carbon’, see Kainth and Melin (2023).
References
Kainth, D. and L. Melin (2023) Adding Conditional and Unconditional Probabilities to the SSP/RCP Scenario Analysis Framework, SSRN paper.
Rebonato, R. and D. Kainth (2023a) Climate Scenario Analysis and Stress Testing with Probabilities: A Modular Approach, EDHEC-Risk Climate Impact Institute working paper.
Rebonato, R. and D. Kainth (2023b) Unconditional Probabilities for Climate Stress Testing via Indirect Elicitation, SSRN paper.
Sunstein, C. (2005) Laws of Fear: Beyond the Precautionary Principle (The Seeley Lectures), Cambridge University Press.